My data suck and so do yours!
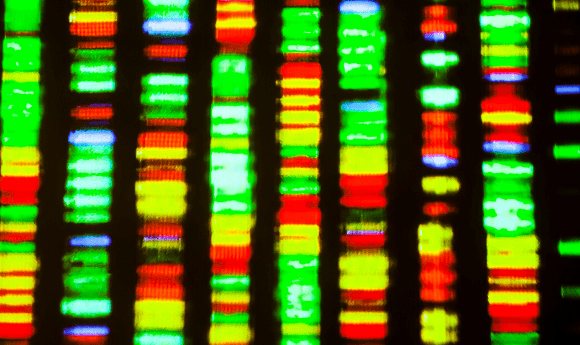
Many microbiome studies utilize DNA sequencing instruments to gather observational data about the proportions of different nucleic acid molecules in a sample. The sequencer is like a microphone in that it is a tool to observe signal and noise. Before DNA can be sequenced, a sample undergoes a dizzying series of processing steps. Each piece of a protocol can introduce noise. These steps can fundamentally alter the outcome of any analysis and need to be well understood if we are to be able to account for the noise and uncover any useful biological signals.
Frequently, we first need to extract DNA. To do so, we crack open the cells in our sample, known as cell lysis, either by chemical or mechanical means. The extraction procedures bias what cells lyse and can damage the nucleic acid molecules, altering the observed frequencies downstream. If our goal is to perform long-read sequencing, we need to ensure that the chromosomal DNA is not too extensively fragmented, while for short read instruments, the long DNA fragments need to be sheared into shorter fragments. When the cells are lysed, many other molecules, including enzymes and other proteins, also get released and can remain biochemically active, further influencing the proportions of nucleic acid molecules. Often, the amount of DNA from a sample is relatively small, so protocols may use PCR to exponentially copy the DNA fragments. PCR relies on enzymes to split apart double-stranded DNA, copying each strand. Sometimes the DNA falls off the enzyme in the middle of a copy, allowing the enzyme to start copying a different piece of DNA – these resulting molecules are aptly called chimeras. Depending on the sample type, or the performance of the DNA purification, a sample may even have small molecules that disrupt the biochemical process by inhibiting the ability to copy some fragments. Finally, the reactions performed in a protocol are frequently done in parallel on many samples arrayed on multi-well plates, for which robots are used to maintain precise control over reagent volumes. However, the robots can also exacerbate well-to-well contamination through splash effects, which can result in major errors if the starting DNA concentration differs substantially from well-to-well – this is something that can occur in human samples for example if skin and stool samples are plated together.
This is by no means an exhaustive list of the steps involved in generating microbiome sequencing data, and all steps involved can introduce bias and noise. Each step is imperfect, and although labs often employ quality checks, these do not necessarily ensure that the resulting sequence data will be truly reflective of the starting proportions. In all of these steps, the chemicals used can be subtly contaminated, the machines or instruments can silently break, and with techniques like mass spectrometry even environmental factors such as atmospheric pressure and relative humidity may shape the resulting data.
In an ideal world, when you provide your DNA to a sequencing machine it should represent the proportions in the original data; but of course, due to the errors introduced through the many potential biases, it will not. Additionally, the sequencing platform itself also introduces error. Machines such as those produced by Illumina (CA, USA) rely on chemicals that emit flashes of light, where the color of the light (or absence of light) denotes a particular nucleotide. The light is weak, so the instrument attempts to cluster molecules together first. If the molecules are highly similar, as is the case with PCR amplicons, it’s important to introduce artificial nucleotide diversity to help the instrument differentiate light sources. The light is captured by high performance charged coupled devices, similar to the one that sits in your cell phone for taking pictures, and these flashes of light are decoded into the ATGCs we can use for analysis. Instruments attempt to measure the quality of these “base calls” in the sequencing process based on how ambiguous the signal for a particular color is. Critically, the reported qualities are an estimate of the error with sequencing, and not necessarily reflective of error in the upstream protocol. The error profiles of instruments can vary, requiring validation experiments for new instruments. It’s not unusual for instruments to exhibit guanine-cytosine (GC) content bias; Illumina instruments tend to undercall these sequences. An error rate of 0.1% is considered pretty good, even though it can represent millions of miscalled nucleotides in a given run.
Fecal sample collection for clinical microbiome research
Daniel McDonald and Jack Gilbert (both University of California, San Diego; CA, USA) discuss the challenges faced during fecal sample collection and highlight important factors to consider when planning a fecal study.
Despite all these existing potential sources of bias, once the data are in-hand we have to work with it, and this is where an analyst begins to clean it up. To minimize sequencing costs, many samples are generally run together, in which the DNA from each sample is assigned a nucleotide barcode that allows us to figure out which sample a DNA molecule belongs to. However, sequencing errors can occur anywhere, including the barcodes, which are therefore specifically designed to tolerate error, to avoid mis-assignment. Overall, sequences with poor quality may be truncated or thrown out, and those containing known artificial constructs added for sequencing are often removed. For amplicon data, it may be possible to correct some of the error introduced by the sequencer with methods like DADA2 and Deblur. Unfortunately, analogs for metagenomic or metatranscriptomic sequencing data have not yet been developed.
At this point, it is best to assume that the data are tentatively good. But, how do you know? With so many opportunities for the introduction of noise, how can you be confident the sequences are reflective of your biological system and not an artifact or an unfortunate mishap with a sneeze from a technician? Sources of error in your data are a reality that must be openly acknowledged, not swept under a rug; all data suck. Thankfully, the microbiome field has put a large effort into open access data practices, and reasonable technical standards for protocols and study variable aggregation. As a result, direct comparison of your data in the context of similar datasets from an existing study can be performed to determine whether your samples are unusual or show unusual trends. Similarly, formal methods for community composition sourcing exist (e.g., SourceTracker), and can be used for another unfortunate reality: sample mislabeling. Introducing standard microorganisms or DNA into your pipeline to determine if they are fundamentally altered in terms of abundance or proportion over the workflow can also help to provide a benchmark for quality assurance.
While many clinical and industrial communities are searching for standard protocols to handle microbiome data, such as those provided by the Earth Microbiome Project available through Qiita and QIIME, there are still concerns about the variability in the quality of bioinformatic analysis. This concern is legitimate as too few people are trained in the statistical approaches needed to properly analyze data, which introduces the ultimate bias for your data interpretation, compounding existing data errors. There are many sources of noise and, depending on the questions being asked, this background can outweigh true signals manifesting as false positives or negatives. At the end of the day, your best ally is first principles. If your data smell bad, stop, investigate, resolve and then proceed.