Deep learning improves de novo protein-binder design
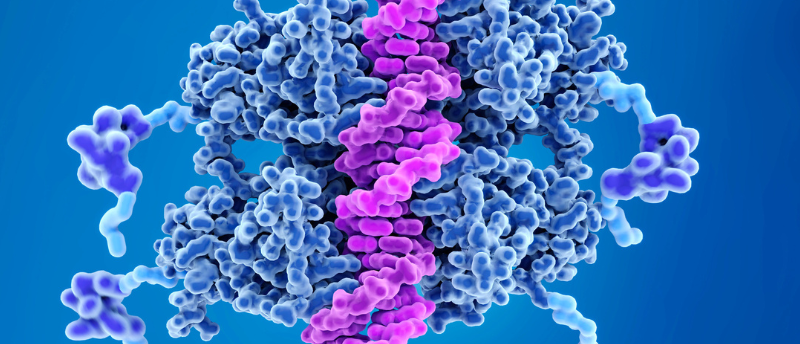
Using deep learning to enhance physical models for protein-binder design increases the target binding success rate.
Researchers at the University of Washington (WA, USA) and Ghent University (Belgium) combined physically based methods with AlphaFold2 or RoseTTaFold to improve de novo protein-binder design. They report a nearly 10-fold improvement in binding success compared to the original energy-based method.
Knowing a protein’s chemical structure and using this to identify or design proteins that can bind to them is key to generating therapeutic candidates and diagnostics. However, “the search space for proteins is enormous,” commented co-author Brian Coventry (University of Washington). For example, a protein made of 65 amino acids, with 20 different amino acid choices at each position, results in 6520 binding combinations – more than the estimated number of atoms in the universe!
In this study, the researchers used deep-learning methods to improve existing energy-based physical models in de novo protein design, designing high-affinity protein-binding proteins from target structural information.
Computer models nanoparticle–protein interactions
Researchers at the University of Michigan have trained a deep learning model to predict molecular interactions, which could revolutionize drug discovery and design processes.
They used machine-learning software tools AlphaFold2 or RoseTTaFold to assess the probability that a designed sequence adopts the designed monomer structure and that it binds to the target protein as intended. Their approach resulted in a nearly 10-fold increase in the success rate of a designed protein binding with its target compared to the original method, which was verified in the lab.
They used yeast surface display binding data for their modeling and produced synthetic genes of around 80,000 designs. Yeast cells were then sorted into those that displayed the proteins and those that didn’t.
“We showed that you can have a significantly improved pipeline by incorporating deep-learning methods to evaluate the quality of interfaces where hydrogen bonds form or from hydrophobic interactions,” explained Nathaniel Bennet (University of Washington), the first author of the study. “This is as opposed to trying to exactly enumerate all of these energies by themselves.”
Although their method improves upon currently available methods of protein-binder design, the success rates among the targets in this study remain low, at less than 1%, and in some instances, no binder was identified for a protein target, including Site 2 of interleukin-2 receptor-α.
“We went up an order of magnitude, but we still have three more to go,” concluded Bennet. “The future of the research is to increase that success rate even more and move on to a new class of even harder targets,” for example, viruses and cancer T-cell receptors.