Test tube brains
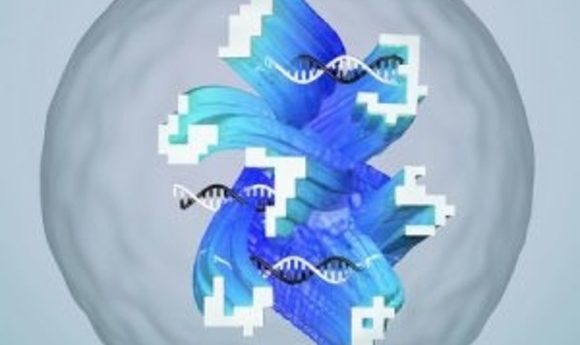
In vitro artificial neural network is able to recognize complex molecular information.
Conceptual illustration of a droplet containing an artificial neural network made of DNA that has been designed to recognize complex and noisy molecular information, represented as ‘molecular handwriting.’ Credit: Olivier Wyart.
Scientists at the California Institute of Technology (CA, USA) have developed an artificial neural network in a test tube that is able to solve a machine learning problem, by correctly identifying handwritten numbers. The study, published in Nature, demonstrates the growing capacity of AI in synthetic biology to perform increasingly complex tasks.
The experiments were performed in the lab of Lulu Qian, who is co-author of the paper.
“Though scientists have only just begun to explore creating artificial intelligence in molecular machines, its potential is already undeniable,” says Qian. “Similar to how electronic computers and smart phones have made humans more capable than a hundred years ago, artificial molecular machines could make all things made of molecules, perhaps including even paint and bandages, more capable and more responsive to the environment in the hundred years to come.”
Artificial neural networks are simplified mathematical models of the neurons in the human brain. They replicate the function of their biological counterparts to process complex information. Qian’s lab’s main goal is to program intelligent behavior with neural networks made of DNA.
“Humans each have over 80 billion neurons in the brain, with which they make highly sophisticated decisions. Smaller animals such as roundworms can make simpler decisions using just a few hundred neurons. In this work, we have designed and created biochemical circuits that function like a small network of neurons to classify molecular information substantially more complex than previously possible,” explained Qian.
To test the capabilities of the networks they had created, first author Kevin Cherry decided to see if they could pass a classic machine learning test; recognizing human handwriting. They demonstrated that the artificial neural networks made out of DNA sequences could carry out certain chemical reactions to identify molecular handwriting.
Molecular handwriting differs from normal handwriting in that each number is made up of a unique set of DNA sequences each assigned to represent a pixel in a 10×10 pattern. These strands are mixed together in a test tube.
“The lack of geometry is not uncommon in natural molecular signatures yet still requires sophisticated biological neural networks to identify them: for example, a mixture of unique odor molecules comprises a smell,” says Qian.
When given one of these molecular numbers, the artificial neural network is able to classify it into one of nine categories representing the numbers 1–9.
Cherry built upon this principle to develop a more complicated system whereby once a number is recognized the DNA would emit fluorescent proteins to indicate the number.
The team plans to develop their networks further; hoping that they will one day be able to form memories. This would allow the networks to perform even more complicated and varied tasks.
“Common medical diagnostics detect the presence of a few biomolecules, for example cholesterol or blood glucose,” concluded Cherry. “Using more sophisticated biomolecular circuits like ours, diagnostic testing could one day include hundreds of biomolecules, with the analysis and response conducted directly in the molecular environment.”