Anne Carpenter on artificial intelligence in the cell imaging field
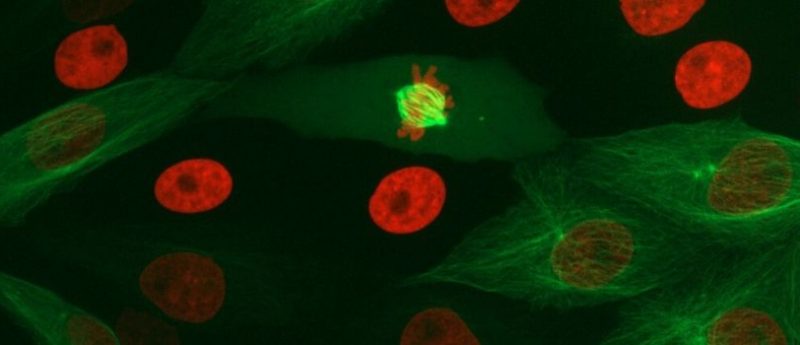
At ASCB 2019, BioTechniques sat down with the Broad Institute’s Anne Carpenter to discuss her work in cell imaging software and how artificial intelligence is revolutionizing the field.
Anne Carpenter is an Institute Scientist and Merkin Institute Fellow at the Broad Institute of Harvard and MIT. Her research group develops algorithms and data analysis methods for large-scale experiments involving images. The team’s open-source CellProfiler software is used by thousands of biologists worldwide (www.cellprofiler.org). Carpenter is a pioneer in image-based profiling, the extraction of rich, unbiased information from images for a number of important applications in drug discovery and functional genomics.
Carpenter focused on high-throughput image analysis during her postdoctoral fellowship at the Whitehead Institute for Biomedical Research and MIT’s CSAIL (Computer Sciences/Artificial Intelligence Laboratory). Her PhD is in cell biology from the University of Illinois, Urbana-Champaign. Carpenter has been named an NSF CAREER awardee, an NIH MIRA awardee, a Massachusetts Academy of Sciences fellow (its youngest at the time), and a Genome Technology “Rising Young Investigator”.
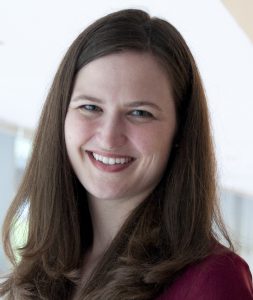
Can you tell us a bit about yourself and your institution?
My name is Anne Carpenter and I am at the Broad Institute of Harvard and MIT (MA, USA). The Broad is a nonprofit academic institution that focuses on technology and collaboration. This means that we have a lot of software engineers, high-throughput screening instrumentation and collaborations among interdisciplinary scientists, which allows us to take on projects that are larger scale than that of a typical individual or academic lab.
Can you tell us about your presentation coming up on Sunday?
I will be focusing on several really interesting biological stories where we have uncovered more in images than biologists would have expected. For example, it’s difficult to tell what stage of the cell cycle cells are in when looking at bright-field images. Aside from some very obvious telophase, you generally can’t tell whether the cell is in G1 or G2 and whether it’s duplicated DNA or not.
We discovered that if you take the bright-field and dark-field images of unlabeled cells, there is sufficient information to predict the amount of DNA that each cell has as well as some phases of mitosis. We can also tell the difference between a cell that’s in G1 versus G2 versus prophase versus metaphase and onward. This was pretty unexpected at the time.
Originally, we did the experiment with classical machine learning. Then we subsequently did it with deep learning, to provide greater resolution. Now, we are going to test all kinds of different disease scenarios to see if this is true across the board.
We want to look at whether we can detect for example leukemia in unlabeled cells. Leukemia is an example where, typically, pathologists are required to use specific antibodies that label specific biomarkers. It took years to figure out which biomarkers you label in order to tell whether a patient’s leukemia is responding to chemotherapy or not.
Now we have this, we need to use these antibodies to label the cells, and these panels can be anywhere from four to ten different antibodies. Once again, we discovered that if you just look at the bright-field and dark-field images alone, the cell’s internal structure is telling you enough about its state that we can distinguish leukemic cells from non-leukemic.
- New AI tech launched to drive drug discovery
- Artificial intelligence set to revolutionize cell imaging methods
- New technology provides insight into cell communication
Can you tell us a bit more about the machine- and deep-learning techniques you are using to uncover this information?
Deep learning is definitely causing a revolution in the computer vision field and in a lot of commercial applications, but now it’s coming to biology as well. Typically, deep learning extracts features that humans may not have considered looking for. You can look at so many different combinations of features of cells that it can go beyond what we are able to articulate and engineer an algorithm to specifically measure. Letting deep learning algorithms loose on images is an unbiased way of taking the raw image data and converting it into a feature space that can go beyond what we can see by eye.
Can you tell us more about the quantification tools that you have developed in your lab?
Our original tool is CellProfiler. I wrote the first version of it 16 years ago, so it’s now quite a mature project and used by thousands of biologists around the world. It’s capable of handling high throughput experiments, which is why we originally wrote it, but some of the same things that make it robust and reliable for high throughput experiments also make it very handy for small-scale experiments. In fact, the majority of our users are doing small-scale experiments, where they want to quantify the results of what they see by eye.
What challenges did you encounter when developing those software tools?
I’d say the biggest challenge for software for biologists is funding. People are often surprised to learn that both CellProfiler and ImageJ, which are the two most popular open-source softwares for image analysis, both only have around one software engineer working on them over the past decades.
You would think there’s a team of dozens that support these projects but it’s actually quite minimal, and even that one engineer is often hard to find. I think it’s really important to consider that, for all other domains of biological software, it doesn’t take much support to keep these projects going. They pay themselves back probably a hundred-fold in terms of how much time they save all the researchers that make use of these different software packages.
“I don’t think there are any fundamental stumbling blocks in this space. There are a lot of bright people who are interested in this problem, who are beginning to work on it. It’s just a matter of letting people’s research unfold because these next few years are going to be pretty exciting.”
Is there any information that you think remains locked away in cell images and image data that we can’t currently access due to limitations in the software?
We’re definitely just scratching the surface of what is possible to extract in images. For the most part, biologists have moved from a microscopy experiment being purely qualitative and most people do quantify the phenomena that they see. However, I would say the vast majority only quantify the phenomena they already see by eye. I think, we should be seeing a shift towards more biologists using microscopy as a data source to look for things that they can’t necessarily see, but are nevertheless robust and reproducible.
Because we’re just starting to do this kind of an experiment, I suspect there are tremendous improvements that can be made in the kinds of features that are extracted. Once a computer tells you that a set of patient cell lines A is different from B, the next question that biologists have is how are they different? That’s a question that is actually a little difficult to answer at times.
There are various methods of taking the results of a deep learning model and interpreting them and understanding the mechanism or the meaning of them. I think that’s a crucial area for improvement so that when the computer does find interesting and unexpected things in images, we can translate that into some kind of deeper biological understanding of the system.
Beyond funding, what needs to change in the field to be able to get to that next step of interpretation of the data?
I don’t think there are any fundamental stumbling blocks in this space. There are a lot of bright people who are interested in this problem, who are beginning to work on it. It’s just a matter of letting people’s research unfold because these next few years are going to be pretty exciting.
“It’s best if computational advancements come from a deep biological need.”
Where do you see the field heading in the next 5 years?
The current version of CellProfiler, for example, uses classical image processing algorithms. We are in the middle of this transition where within a few years biologists are going to be able to drag-and-drop their images into a web app and the software will automatically recognize a variety of structures within the cell without any training or tweaking or understanding how the image processing is working. If we can train models that are generalizable enough, we should be able to get to the point where image analysis becomes quite a lot easier for the biologists.
What has been the main driver in your career path?
The progression of my career, transitioning from a pure cell biologist and microscopist into the computational field, has been primarily driven simply by following the next step for various projects that I personally was working on. In general, it’s best if computational advancements come from a deep biological need. I would say that probably defines my career progression up until now.
Do you have any advice for early career researchers starting out in a similar field?
I think as you’re developing in your career, it’s great if you can identify an area that is underpopulated, is useful to your research and really makes you excited. I think following the combination of the biological need and your interest level can lead you to create a vision of the future that you can then pursue for many decades.