RNA sequencing: as accurate as once believed?
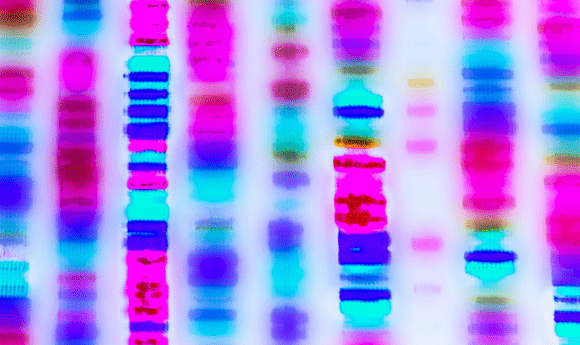
Once hailed as the most accurate way to measure gene expression, RNA sequencing’s reproducibility has now been called in to question as a source of potential bias is highlighted.
A popular technique in a geneticist’s toolbox, RNA sequencing has many applications. Allowing researchers to quantitively measure gene expression in organisms of all species, the technique can give scientists a greater understanding of what is happening in a cell at any time and offers vital information about the function of specific genes. With potential roles in drug discovery, diagnostics and gene identification, the uses of the method seemed unlimited.
Affirming the accuracy of RNA sequencing
Back in 2014, three articles were published in Nature Biotechnology as part of a project that aimed to assess the performance of next-generation sequencing platforms and evaluate the advantages and limitations of RNA analysis. Funded by the US FDA (MD, USA) and known as the Sequencing Quality Control (SEQC) study, it tested three commonly used RNA-sequencing platforms for reliability, accuracy and information content and hoped to define the scope of the platforms as well as find sources of variation.
Across the study, more than 1 billion nucleotides of data were generated by each of the three institutions involved. They also examined the technologies used by 30 different RNA-sequencing labs and the biochemical methods of hundreds of individual researchers. Overall, they appeared to find that RNA can be extracted and analyzed accurately across institutions and that data remain reliable even when the genetic samples have been severely degraded.
The results were thought to reassure the research community, clinicians and patients alike that RNA sequencing is accurate and reliable. “It seems very likely that decisions about patient care are going to be influenced by genomic data, derived from sequencing both RNA and DNA from patient samples, and we now know the extent to which these sequence-based analyses can be relied upon within a given laboratory or from laboratory to laboratory,” commented one of the study leaders, E. Aubrey Thompson of the Mayo Clinic in Florida (FL, USA).
The issue of reproducibility
Fast-forward 5 years and we are currently in the middle of the so-called reproducibility crisis, where more and more studies are being found to be difficult or even impossible to reproduce. In 2015, it was estimated that in the USA alone, approximately $28 billion was spent on preclinical research that has since been found to be irreproducible. Aside from the economic costs, the growth of irreproducible research has led to delays in drug development, stalled the discoveries of life-saving therapies and increased the demand on currently available resources.
Reproducibility remains a challenge that all researchers must bear in mind when designing an experiment and deciding on which techniques to use; and methods shown to be reliable, such as RNA sequencing, are often a go-to for many. However, a recent metanalysis from a group at Tel Aviv University (Israel) suggests that a technical bias that occurs during the analysis of data taken from RNA sequencing could have led to a widespread misinterpretation of data and a large volume of false results.
- HPV-RNA Seq: a powerful diagnostic technique for cervical cancer?
- RNase: comparing thee to snow on a winter’s day
- Fake news, the reproducibility crisis & politics – science communication with Gareth Mitchell
Irreproducibility in RNA sequencing?
Following the analysis of 35 publicly available RNA-sequencing data sets, the researchers noticed that certain sets of genes repeatedly showed changes in gene expression. The data were taken from a combination of human and mouse studies that had been published in recent years and covered a diverse set of biological processes. In 30 out of the 35 sets, they found that gene sets that were either particularly long or particularly short displayed the most changes in levels of gene expression. The majority of the short genes identified encode proteins that form part of the ribosome, the organelle responsible for translating mRNA into proteins, and the recurrent long genes identified were found to encode for proteins involved in the cell’s extracellular matrix.
Upon further investigation into the puzzling pattern, the researchers found that it stemmed from an experimental artefact, rather than any innate biological response to a trigger. When comparing replicate samples from the same biological condition, they found that the pattern was due to a technical bias that appeared to be coupled with the length of the gene. In addition, the detected length bias, when combined with flaws in any statistical analysis, could lead to the false labeling of biological functions as cellular responses, in particular those related to the ribosome or extracellular matrix. This bias was found not to be corrected for in many commonly used data-normalizing methods and therefore is likely to have been included in many more data sets.
The effect, known as the sample-specific length effect, has been described previously in the literature and many methods-oriented scientists are actually aware of the issue. The more surprising result of the study is the notion that many researchers are not actively addressing it, as shown by the high percentage of data sets where it has not been corrected for.
Never underestimate the importance of statistics
Although worrying at first glance, the results are not as negative as they appear. As well as identifying the bias, the researchers also describe how to overcome it and remove it from the data, allowing for any false results to be filtered out while maintaining the biologically relevant ones. By considering the length of the gene as a sample-specific covariate, the number of false positives can be markedly reduced while the true results remain.
The exact factors that underplay this sample-specific length effect are not fully understood and further studies into this are required. The authors recommend that researchers be on the lookout for this bias in their own studies and that they implement the suggested data-normalization methods as default steps in their standard practice for analysis of RNA-sequencing data.
Michael Love, a biostatistician from the University of North Carolina-Chapel Hill (NC, USA) who was otherwise not involved with the study told The Scientist, “[this paper is] a really nice demonstration of how important it is to do quality control checking.” He also noted that there are other biases that are known to affect RNA-sequencing data, such as GC-content bias, and these also need to be accounted for consistently across research teams.
If anything, the study highlights that not all techniques should be accepted as 100% reliable as there is always the potential for bias. As concerns over the reproducibility crisis grow across all areas of scientific research, this work reinforces the need for accurate statistical analysis, no matter how well supported the accuracy of the underlying technique may be.