Analyzing aberrant DNA methylation for a new generation of cancer diagnostics
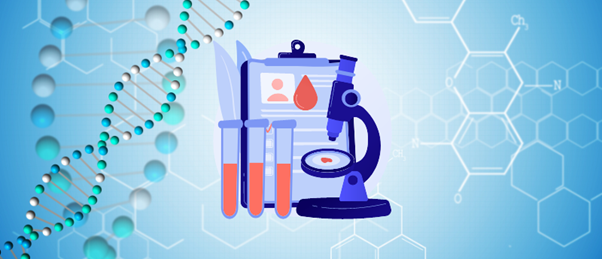
Using aberrant DNA methylation patterns, scientists have expanded our arsenal of diagnostics for difficult-to-detect cancers.
A team of researchers led by Ben Ho Park (Vanderbilt University Medical Center; TN, USA) has developed a novel multicancer early detection (MCED) diagnostic that utilizes aberrant methylation patterns to detect numerous cancers from a single blood biopsy. This test could expand our ability to detect early-stage cancers and cancer types for which the development of screening tests has so far proved elusive. The team presented the data from this study today at the American Association for Cancer Research’s Annual Meeting (AACR; April 14-19 2023; Orlando, FL, USA).
Early detection of cancers is a vital prognostic factor in cancer; however, screening technologies are currently only available for a limited number of cancer types. What’s more, “the majority of cancer deaths are from cancers for which there are no screening methods,” Park notes. A persistent challenge in the development of diagnostics for a broader range of cancers is that many types shed very little cell-free DNA (cfDNA) into the blood, meaning that technologies need to be highly sensitive.
Examining alternative approaches to address this solution, Park and his team decided to focus on the aberrant DNA methylation that often drives cancer initiation and maturation. These aberrations include both differential methylation patterns that are consistent across cancer types and more tissue-specific aberrant methylation patterns.
Aiming to utilize this characteristic of cancer, the team has set out to develop a genome-wide methylome enrichment platform, which sequesters cfDNA in the blood, avoiding chemical or enzymatic treatment. The platform facilitates the sequencing of the sample’s entire methylome without sequencing the genome. The sequence obtained can then be analyzed by a machine learning classifier.
Top tips for maximizing your time at AACR 2023
We share our advice on making the most of attending a conference and our top picks of sessions being presented at AACR’s Annual Meeting 2023.
The team utilized a cohort of approximately 4000 newly diagnosed, treatment-naïve cancer patients – 50% of whom had early-stage cancers – with age- and gender-matched non-cancer controls. Using a subset of 1903 samples from this cohort, the team tested their machine learning classifier, first training it on 80% of the samples and then testing it on the remaining 20%. They evaluated the performance of the classifier using the metric of the area under the curve (AUC), with which a score of one represents perfect discrimination.
When assessing the performance of the MCED for detecting all cancers, the test delivered an AUC of 0.94, which is broken down into 0.92 and 0.95 for stage I and stage II cancers respectively. For detecting individual cancers, AUC ranged from 0.89 to 0.99, with the five low-cfDNA-shedding cancers averaging an AUC of 0.91.
Discussing the implications of these results, Park stated, “This platform allowed for a higher signal-to-noise ratio and led to increased performance in the more challenging applications where the cfDNA burden is the lowest. Cancers that shed a high amount of cfDNA had the best performance, but even cancers that are typically difficult to detect with cfDNA assays were detected with high performance in this interim readout. At this early stage in development, the robust detection of early-stage and low-shedding cancers with this genome-wide methylome enrichment platform is very promising.”