AACR22: Artificial intelligence predicts side effects of combination therapies
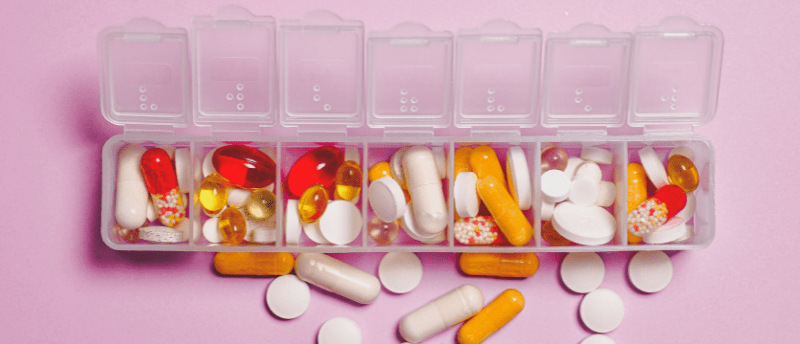
A machine learning model shows promise in predicting potential adverse effects from new drug combination therapies, a method commonly used to treat various cancers.
Researchers at the Cancer Center Amsterdam (Netherlands) report their preliminary findings using artificial intelligence to predict the adverse effects of combination therapies. The findings were presented by graduate student Aslı Küçükosmanoğlu at the AACR Annual Meeting 2022 (8–13 April; New Orleans, LA, USA).
It has become increasingly common to use combination therapies to treat different cancer types and other diseases, as this can reduce the risk of treatment resistance and improve its efficacy. However, in many cases, this involves adding multiple drugs to an already extensive list of medications, which can make it challenging to predict potential adverse effects. Additionally, clinical trials rarely take into account the medications that patients are using outside of the trialed treatment regime.
“Patients seeking treatment commonly use four to six medicines daily, making it difficult to decide whether a new combination therapy would risk their health,” explains Bart Westerman, the senior author of the study. “It can be hard to assess whether the positive effect of a combination therapy will justify its negative side effects for a certain patient.”
The research group developed a machine learning model to predict potential adverse effects from new drug combinations. “Our approach can help us to understand the relationship between the effects of different drugs in relation to the disease context,” explains Westerman.
Suppressing the immune system improves pancreatic cancer treatment
Researchers find that blocking a key pathway in the immune system more than doubled survival rates in a pancreatic cancer mouse model.
They collected data from the U.S. Food and Drug Administration Adverse Event Reporting System (FAERS), which contains over 15 million records of side effects. These were grouped into frequently co-occurring events using a method called dimensional reduction to simplify the analysis and strengthen the associations of a drug and its side-effect profile.
This data was then fed into a type of machine learning that imitates how brains make associations between data called a convolutional neural network algorithm. The machine learning platform was trained using adverse events from individual therapies so it could identify patterns between drugs and their side effects. These patterns were encoded into a string of 225 numbers between 0 and 1 to simplify calculations, referred to as “latent space”. This string of numbers can then be decoded back to the original side-effect profile of the drug.
The model was tested using unseen adverse event profiles of combination therapies, called the “adverse event atlas” to assess if the AI model could decode new profiles using latent space descriptors. The researchers report that the model did recognize patterns and decode them to the side-effect profiles of individual drugs in the combination therapy.
“We were able to determine the sum of individual therapy effects through simple algebraic calculations of the latent space descriptors,” explained Westerman. “Since this approach reduces noise in the data because the algorithm is trained to recognize global patterns, it can accurately capture the side effects of combination therapies.”
To validate the model, the researchers compared the predicted side-effect profiles from combination therapies to those observed in the clinic using data from FAERS and the U.S. clinical trial database. The model was able to predict the profiles for commonly used combination therapies, and the researchers are now developing a statistical method to quantify the accuracy of the model before it can be used in clinical practices.
Another aspect to consider in combination therapies are side effects that occur only when drugs are combined, so are not seen in individual side-effect profiles. The researchers used the additive patterns identified by their model to differentiate additive side effects from synergistic side effects of various drug combinations. Westerman explains this could improve understanding of side effects that may arise when complex adverse event profiles intertwine.
“Given that the landscape of drug interaction is highly complex and involves many molecular, macromolecular, cellular, and organ process, it is unlikely that our approach will lead to black-and-white decisions,” says Westerman. “The adverse events atlas is still in the proof-of-concept phase, but the most important finding is that we were able to get snapshots of the interplay of drugs, diseases, and the human body as described by millions of patients.”