Computer model identifies cancerous driver mutations
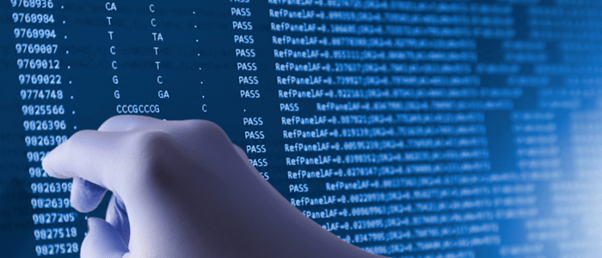
Researchers have developed a computer model to scan the entire genome of cancer cells to identify driver mutations, which could help researchers find novel drug targets.
Cancer is only driven by some DNA mutations; however, cancer cells have thousands of different mutations present, making it difficult to differentiate harmful driver mutations from neutral passenger mutations. Being able to distinguish between these mutations could lead to improved identification of drug targets. To assist in this endeavor, researchers at MIT (MA, USA) have developed a computer model that can scan the entirety of a cancer cell’s genome to identify mutations that could be contributing to tumor growth.
As some regions of the genome experience a higher frequency of passenger mutations, the harmful mutation signals can be drowned out, meaning that predicting the driver mutations is challenging. As a result, at least 30% of cancer patients have no detectable driver of cancerous mutations, which makes it difficult to guide treatment.
Some previously identified mutations, like the epidermal growth factor receptor in lung tumors, have led to successfully targeted, specific drugs; however, this is a protein-encoding gene, which represents around 2% of the genome. Currently, tools to identify driver mutations in the remaining 98% of the genome are lacking, which has made it challenging to identify which of these mutations contribute to cancer.
Bioimage analysis: has deep learning changed the game?
We spoke to Beth Cimini, an analyst at the Broad Institute of MIT and Harvard (MA, USA), about her role at the institute and how bioimage analysis has evolved in recent years due to COVID-19 and the application of deep learning.
In order to address these issues, the researchers designed a deep neural network, a type of artificial intelligence, to scan cancer genomes for unexpectedly frequent mutations. To do so, they trained the model on 37 different types of cancer to determine a background mutation rate with data obtained from the Roadmap Epigenomics projects and the Pan-Cancer Analysis of Whole Genomes.
“We created a probabilistic, deep-learning method that allowed us to get a really accurate model of the number of passenger mutations that should exist anywhere in the genome,” says Maxwell Sherman, the first author of this study. “Then we can look all across the genome for regions where you have an unexpected accumulation of mutations, which suggests that those are driver mutations.”
The resulting data analysis produced a map of expected passenger mutation rates across the genome, allowing the expected rate to be compared to the observed mutation count anywhere in the genome.
Using this model, the researchers identified additional mutations that may be contributing to tumor growth in 5 to 10% of cancer patients. Being able to identify these mutations could help doctors decide the most suitable treatment options for individual patients.
“The really nice thing about our model is that you train it once for a given cancer type, and it learns the mutation rate everywhere across the genome simultaneously for that particular type of cancer,” explains Sherman. “Then you can query the mutations that you see in a patient cohort against the number of mutations you should expect to see.”
The researchers used this model to investigate different cancer mutations, including common driver mutations such as the BRAF mutation, which is linked to melanoma. They found that this mutation is also present at varying frequencies in other cancers and is linked to tumor progression, including pancreatic, liver and gastroesophageal cancers. “That says that there’s actually a lot of overlap between the landscape of common drivers and the landscape of rare drivers. That provides opportunity for therapeutic repurposing,” explains Sherman.
Sherman concludes: “These results could help guide the clinical trials that we should be setting up to expand these drugs from just being approved in one cancer, to being approved in many cancers and being able to help more patients.”