Detecting noncoding RNA sequences improves cancer diagnostics
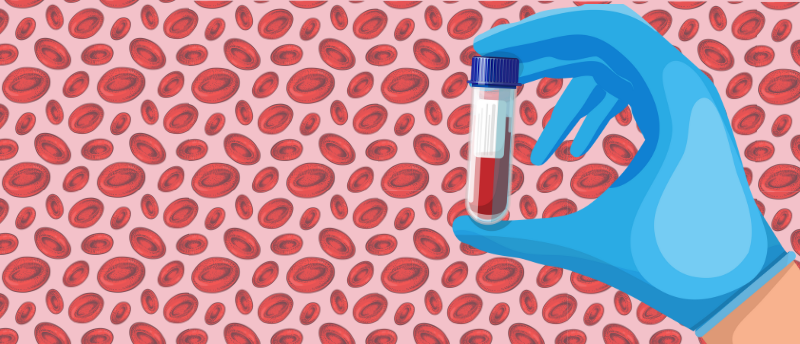
Profiling noncoding repetitive RNA sequences improves the sensitivity of liquid biopsy diagnostic tests for early stage cancers.
As cancer is most treatable in its early stages, developing diagnostic tests that can detect cancer early on is crucial. Liquid biopsies are an emerging non-invasive cancer diagnostic that sequences either RNA or DNA from blood samples; however, tests that are currently available are less sensitive to early stage cancer. To address this, researchers at the University of California, Santa Cruz (UCSC; CA, USA) led by Daniel Kim have leveraged noncoding and repetitive RNA sequences to improve the performance of liquid biopsy tests.
Noncoding RNA, dubbed RNA ‘dark matter’, remains an understudied area of the genome. 75% of the human genome generates noncoding RNA, a substantial portion of which is derived from repetitive elements. Few of these repetitive noncoding RNA sequences are present in the blood of healthy individuals; however, Kim’s research group has previously shown that many repetitive RNAs are secreted out of cancer cells, even at the earliest stages of cancer. This finding suggested that repetitive RNA could be a suitable biomarker for early stage cancer diagnostics.
Many researchers are currently pursuing DNA-based liquid biopsies, but in light of their previous findings, this research team is focusing on harnessing noncoding and repetitive RNA. They developed a cell-free RNA sequencing and analysis platform called COMPLETE-seq, which identifies repetitive noncoding RNAs as well as protein-coding RNA.
Keeping a finger on the pulse of antipsychotic drug detection
Researchers at the University of Surrey have developed a test for monitoring antipsychotic drugs utilizing sweat collected from individuals’ fingertips.
Diagnosing cancer in its early stages is challenging due to the small tumor size, which means the biological signals are low. By detecting both noncoding and coding RNA with COMPLETE-seq, the researchers were able to increase the biological signals available, resulting in a more sensitive diagnostic test.
“When we trained machine learning models for cancer classification, the models perform better when you introduce these repetitive cell-free RNAs as additional features,” explained Kim. “We see higher sensitivity in terms of detecting cancer, so we think that these repeat elements are actually providing a lot of rich cell-free RNA information that people previously hadn’t looked for.”
They trained COMPLETE-seq to detect several cancer types and compared it to their repeat-naïve counterparts. The platform showed promising results for detecting colorectal cancer (91% sensitivity), stomach cancer (86% sensitivity) and lung cancer (93% sensitivity), whilst esophageal cancer had a 56% sensitivity.
The researchers hope to develop a versatile RNA liquid biopsy test that can detect multiple cancers in their early stages in the clinic. Further studies will be needed to generate larger and more diverse cell-free RNA transcriptomic datasets of different cancer types in their early stages to achieve this.