Liesbeth Hondelink and Reidunn Jetne Edelmann on deep learning for cancer research and early career advice for women in STEM
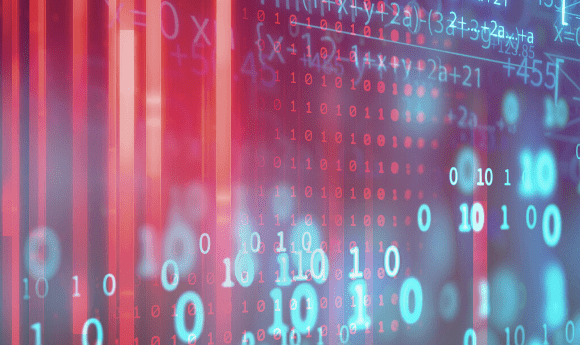
In honor of International Women’s Day, BioTechniques sat down with Liesbeth Hondelink and Reidunn Jetne Edelmann, two women making waves in STEM to, respectively, discuss their recent work developing deep learning algorithms to improve research for stage IV non-small cell lung cancer patients, and discover biologically relevant “high-content” tumor vessels in breast and colon cancer.
Please introduce yourself and your institution
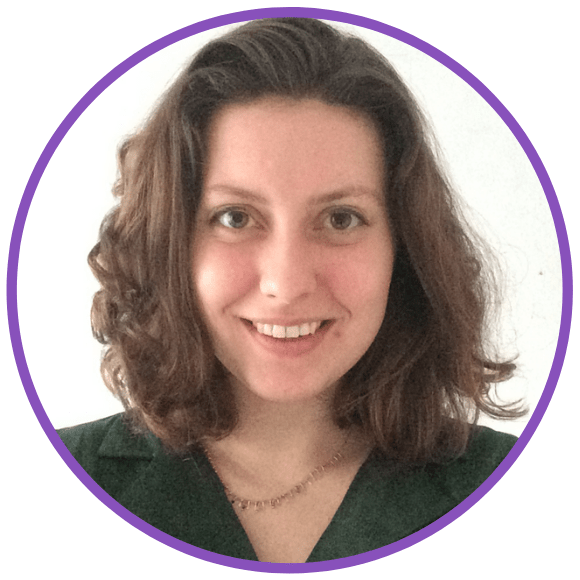
Hondelink: I’m Liesbeth Hondelink. I have recently started working at the Leiden University Medical Center (Netherlands) after graduating from Leiden University on Jan 31, 2020.
I work in the Pathology department as a PhD-candidate in Dr. Danielle Cohen’s research group and my research is mainly focused on stage IV lung cancer. I was affiliated with the Pathology department during my studies and my MSc thesis was conducted with the help of the aiForward program.
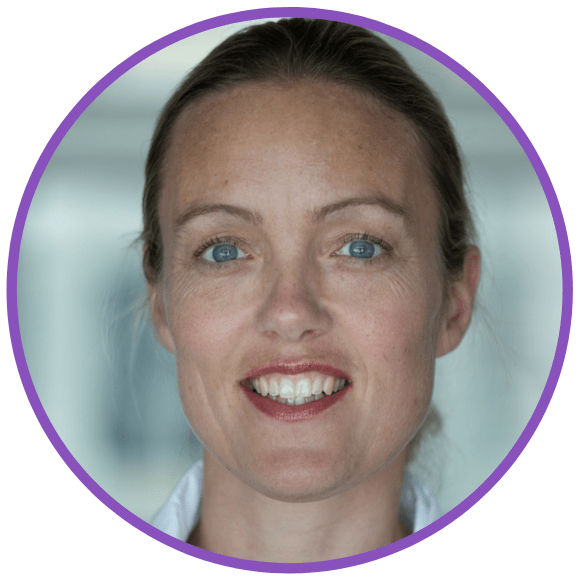
Edelmann: I’m Reidunn Jetne Edelmann. I am an MD PhD affiliated with the Center for Cancer Biomarkers at the University of Bergen (Norway), a Norwegian Center of Excellence established by the Research Council of Norway (Oslo, Norway). The center has a strong record of single-marker studies and multiplexed profiling of tissue samples from several human cancer types, especially breast cancer, with a particular focus on the tumor microenvironment, including the vasculature and immune responses.
Can you tell us about your research?
Hondelink: My research focuses on stage IV non-small cell lung cancer (NSCLC). This is a very common type of cancer and, unfortunately, due to the relatively long symptom-free period, many people are already stage 4 (irresectable and no curative options) when they are diagnosed. Therapy in stage IV NSCLC is therefore aimed at providing comfort and life-prolongation. However, novel treatment options have been discovered recently, which are proving to be a real game-changer for stage IV patients!
Some of these novel therapies are based on the interaction between lung cancer cells and the immune system. If lung cancer cells express PD-L1, they are able to bind to the PD-1 receptor on the T-cells, and these T-cells will then self-destruct or deactivate. For lung cancer cells this is a very lucrative way to survive and keep growing. When we block the PD-L1/PD-1 interaction with immunotherapy drugs (e.g. pembrolizumab of nivolumab), the T-cells are not deactivated and instead attack the tumor, which slows down cancer progression in the patient for a significant amount of time.
However, this PD-L1/PD-1 blockage is much more effective when tumor cells use PD-L1 to hide from the immune system, which not all of the tumors do. Pathologists, therefore, need to investigate whether the tumor expresses any PD-L1 and quantify how intense this expression is. To do this, they use the tumor proportion score (TPS), which involves estimating the percentage of tumor cells staining positive for PD-L1. This percentage estimate has severe consequences, with percentages below a certain cut-off resulting in changed treatment choices.
We know from recent studies that estimating the PD-L1 expression is very difficult for pathologists. If you ask two pathologists what the TPS of a randomly selected patient is, in 20% of cases they disagree to such a degree that a pulmonologist would prescribe a patient a different drug based on each pathologist TPS. Additionally, if you ask the same pathologist to re-score the case, in 10% of cases they will change their mind. Worse still, it’s been shown that extra training does not improve these data.
Therefore, we figured it would be a good idea to develop a computer-mediated scoring system, to help the pathologists score PD-L1 expression in difficult cases, or to assist when two pathologists disagree. We have included approximately 200 lung cancer specimens and a deep learning AI algorithm was created to help quantify PD-L1. So far, our results are very promising and the algorithm scores are comparable to the pathologists.
Edelmann: Our research focuses on the tumor vasculature, a major, and significant, component of the tumor microenvironment. Beside prominent roles in assuring nutrient and oxygen supply, tumor vessels provide soluble factors and surface proteins that regulate immune cell activation and extravasation, tumor cell intravasation and metastatic spread.
Recent progress in vascular biology has identified a set of vascular biology molecular pathways affecting processes of high relevance for tumor biology, such as sprouting angiogenesis, arterio-venous differentiation and extravasation of immune cells. These model-based studies thus suggest yet-to-be-defined functionally distinct vessel sub-types which are expected to display differential associations with outcome.
Endothelial Notch-signaling plays a central role in many aspects of vascular biology. First, Notch signaling is the central coordinator of sprouting angiogenesis, as it coordinates the selection of tip-cells (low in Notch1 signaling activity) and stalk cells (high in Notch1 signaling activity), the number of vessel branches, vessel diameter and arterio-venous differentiation; second, Notch-signaling supports inflammatory activation of endothelial cells, including upregulation of VCAM1, leading to chemotaxis, adhesion and transmigration of immune cells (e.g. t-cells and immunosuppressive myeloid cells), as well as tumor cell intravasation and homing to distant organs; and third, Notch ligands (e.g. jagged1) expressed on endothelial cells can activate Notch receptors on tumor cells, enhancing their aggressiveness and cancer stem cell phenotype.
By annotating Notch1/Jagged1-defined tumor vessel phenotypes, in a human tumor tissue cohort, we expect to uncover novel biologically relevant “high-content” biomarkers. Furthermore, studies will also allow a set of exploratory studies regarding relationships between vessel phenotypes and tumor characteristics that could validate, or dis-qualify, mechanisms that have been proposed based on studies in mouse models. Thus, our project does not only hold the potential for discovery of new biomarkers through a novel vessel annotation approach, but will also constitute a long-awaited mapping of Notch signaling in human tissue.
Earlier analyses of tumor vasculature in clinical samples have generally been of “low-content” nature, restricted to analyses of features such as vessel density, vessel size, endothelial cell proliferation and perivascular status. Our project aims to create a deep learning AI algorithm to annotate “high-content”-defined tumor vessel phenotypes, defined by multiplexed immunohistochemistry, and correlate them to outcome-related end-points as well as to biological characteristics of the tumors, including driver mutation status and tumor immune infiltrates, in different tumor collections.
Deep learning in genomics with Olga Troyanskaya
At ASHG 2019, BioTechniques spoke to Olga Troyanskaya (Princeton University; NJ, USA) about her new deep learning framework for the study of sequences; Selene.
What challenges do you face in your research?
Hondelink: We really struggled to find a suitable platform to build a deep learning algorithm. The most common problem with applying artificial intelligence (AI) to solve healthcare problems is that people either have AI-knowledge or healthcare-knowledge, but usually not both. This makes research on AI-related topics difficult, because you really need expertise on both subjects and finding a way to collaborate with someone so far away from your own profession can be difficult.
Edelmann: The overall hypothesis of the study is that quantification of complex “high-content” vessel features will be superior to standard approaches both with regards to identification of strong biomarkers, and for explorative biology-related studies. Quantification of such features without AI support would be prohibitively time consuming and subject to large errors due to observer limitations.
How has aiForward helped you to overcome these challenges and with other areas of your research?
Hondelink: My MSc thesis was included in Aiforia’s (Helsinki, Finland) global aiForward program. Using their software meant that I did not have to program anything myself, so I could instead focus on the correct annotations. This made our project much more efficient and a lot faster.
Edelmann: Our AI-based approach takes tumor vessel annotation to a new level, with the promise of a more comprehensive characterization of different vessel phenotypes, which has not been seen before. We thus believe that this study can serve as a powerful proof-of-concept study for the general notion that AI-based sample image analyses can be used for analyses which in an unprecedented way allows in situ tissue analyses of complex and molecularly well-defined biology.
Do you have any advice for other women in STEM jobs or research roles, early in their careers?
Hondelink: Pursue what you are truly passionate about and take a lot of initiative! For me, this advice has worked out pretty well: I am truly passionate about improving the prospects for cancer patients, and I definitely took a lot of initiative by stepping out of my comfort zone to try to create a deep learning algorithm.
Edelmann: Remember to build your CV! I think men are often more confident with this early in their careers. Put yourself out there. Remember, it is not always just what you know and what you have done – you have to show it off and let the world know!
What do you think needs to change in order to ensure equality among genders in STEM jobs?
Hondelink: We need to stop nudging girls toward the ‘female’ professions (i.e. teaching, psychology, childcare) and boys toward the ‘male’ professions (i.e. science, engineering, IT) throughout their childhood and education. I experienced this nudging myself and it made me choose a study on the ‘female’ end of my aptitude and interest (Medicine).
We need to have more prominent female role models. Both history books and the media are filled with stories about men in STEM jobs, which can be quite discouraging and can make choosing or continuing a STEM-profession more difficult for women.
Edelmann: I have been very fortunate to grow up in a country with a very strong public-school system, where girls and boys are treated equally and everyone is given the same opportunities. I think it all starts there, at early education level. There is absolutely no reason why gender should play a role in science and to consider that there is one is, in my opinion, utterly unscientific.
Learn more about Aiforia here.