New tool virtually realigns 2D samples to improve 3D tissue analysis
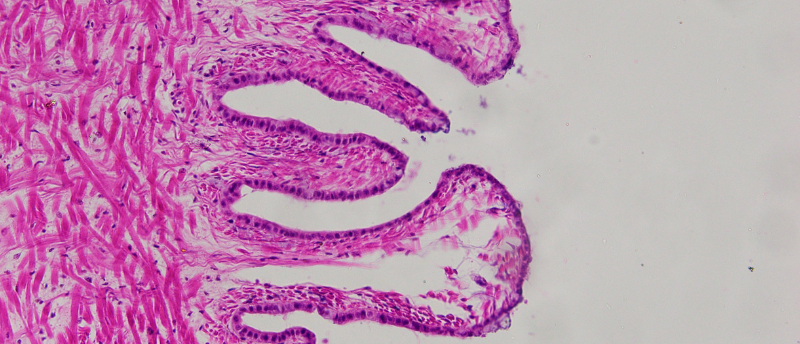
A new tool aligns spatial genomic and histology data from tissue samples virtually, creating a 3D atlas from 2D slices.
Using 2D tissue slices to decipher features of a 3D tissue is computationally challenging and leaves a lot of questions unanswered. A new method called Gaussian Process Spatial Alignment (GPSA) can align spatial genomic and histology data obtained from 2D tissue slices virtually, providing a more in-depth view of tissues. This was reported in a study led by Barbara Engelhardt at Gladstone Institutes (CA, USA).
“Without that third dimension, you can miss a lot of what’s happening in tissue,” commented Engelhardt. “Putting together slices in 3D space should help us begin to answer questions for which 2D data falls short. For instance, what are the precise boundaries of a tumor? Where have immune cells infiltrated the tumor? Where in the tumor would be best to inject a treatment?”
Studying biological tissue often involves slicing the tissue into thin pieces to view under a microscope or carrying out tests to detect the presence of specific molecules. However, due to constraints in time, budget and computational power, the number of slices that can be analyzed is often limited, which makes it challenging to piece together a comprehensive 3D profile of the original tissue. Additionally, slicing can lead to spatially warped samples.
Deep learning improves de novo protein-binder design
Using deep learning to enhance physical models for protein-binder design increases the target binding success rate.
“The first step in going from 2D slice data to a full, 3D picture of the tissue is to computationally reverse warping so that we can realign the slices in virtual space,” explained Engelhardt.
To address this, the GPSA uses a statistical approach consisting of a two-layer Gaussian process. This uses data from the 2D tissue slices and fits the warped 2D slice onto a 3D model of the tissue for the first layer. In the second layer, GPSA attributes data collected from the slice, such as gene expression, to each point within the 3D model. This means it can virtually reverse the spatial warping, resulting in the precise alignment of the tissue slices. GPSA also fills in the spaces between slices with predictions for every point in the tissue, creating a 3D atlas.
“Say you have four slices from different locations in a person’s breast cancer tumor, and for every point on each slice you know which of 20,000 genes are turned on or off,” Engelhardt explained. “GPSA creates a fully query-able 3D atlas where, for any single ‘x, y, z’ coordinate, for any of the 20,000 genes, we can dive in and ask: What genes are on and off at this position in the tumor? And how certain are we in this estimate?”
GPSA’s flexibility is one of the tool’s strengths, enabling researchers to construct tissue atlases from 2D slices of varying sizes, obtained using different technologies and at different scales and resolutions. It can also combine different types of data, for example, information about genes and cellular structure.
Additionally, GPSA can predict how locations within the tissue change over time by applying it to slices taken from the same tissue at different time points. This could be used for studies relating to aging, how illnesses progress and how different tissues develop in a growing organism.
The researchers have also developed a method to determine the number of tissue slices needed, and the locations these slices should be obtained from, to enable GPSA to construct a tissue atlas.
Going forward, the researchers hope “to maximize the insights we can gain from tissue slices, in order to allow researchers and clinicians to deeply query 3D tissues that are well-studied or tumors that are unique to a patient, and ultimately improve healthcare,” concluded Engelhardt.