Pick of the posters: AACR 2024
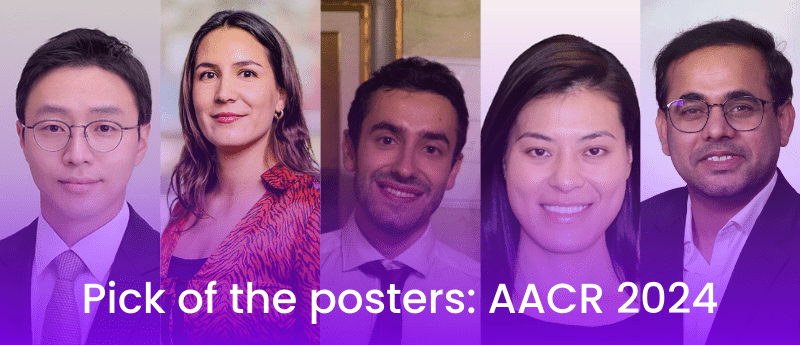
At AACR 2024, we explored the poster hall to pick out the posters that would interest the BioTechniques reader and those we found delivered the most interesting or surprising findings. Get our selections below and let us know your thoughts on our Pick of the posters.
Contents:
- pyVIPER: a fast and scalable toolkit for the identification of dysregulated proteins in tumor-derived single-cell RNA sequencing data
- An isoform resolution transcriptomic atlas of colorectal cancer from long-read single-cell sequencing.
- AI-enhanced spatial proteomics reveals B cells and FOXP3 cells interactions in HPV-positive oropharyngeal squamous cell carcinoma progression.
- Microbial signatures and serum markers of colon cancer in African American patients
- Data-driven discovery of cancer-specific targets for hepatocellular carcinoma using spatial transcriptomics
pyVIPER: a fast and scalable toolkit for the identification of dysregulated proteins in tumor-derived single-cell RNA sequencing data
Luca Zanella
Postdoctoral researcher
Columbia University (NY, USA)
What does your poster present
Our poster presents pyVIPER, a novel computational toolkit for RNA-seq data analysis, based on the VIPER core algorithm for virtual inference of protein activity by enriched regulon analysis, published by our lab (the Califano Lab) in 2016. The VIPER algorithm takes a gene expression matrix as input and transforms it into a protein activity matrix by leveraging a gene regulatory network, thus estimating the activity of each regulatory protein based on the expression of its downstream targets. The approach is very powerful and it can be used to identify Master Regulators, proteins that are aberrantly activated in a disease.
This framework is instrumental for the analysis of gene expression data, especially single-cell RNA-seq data, which suffer from gene dropouts and are extremely sparse. pyVIPER counteracts data sparsity through virtual protein activity estimation using gene sets, thus increasing the signal-to-noise ratio.
pyVIPER is a versatile Python-based toolkit. It is built on top of Scanpy and the AnnData class and has several functionalities for data preprocessing, transformation and postprocessing, along with different tutorials for protein activity-based analysis of single-cell data. Some functions are tailored to cancer research, like OncoMatch, an algorithm for matching tumor samples to models (such as organoids or cell lines) to find the models that best recapitulate the transcriptional state of the original tumor.
Moreover, compared to the previous VIPER implementations, the redesigned pyVIPER core function excels in terms of speed, being faster by several orders of magnitude. This allows upscaling protein activity analyses to larger single-cell datasets comprising several thousands of cells.
Do you have any tips for best practice when using the pyVIPER pipeline or conducting single-cell RNA sequencing analyses?
We have curated a set of tutorials, available on GitHub (https://github.com/alevax/pyviper), which highlight several best practices, including the optimal generation of a gene expression signature, recommendations on how to run the pyVIPER pipeline (for instance when to use one or multiple regulatory networks and how to post-process VIPER-inferred protein activity matrices), and how to elucidate different cellular states in tumor samples.
What do you hope the impact of this poster will be on the field?
I think pyVIPER will provide the community with a set of tools for the computational elucidation of cellular populations and their transcriptional programs in a variety of disease contexts, especially cancer. The advantage of this framework is that it provides highly mechanistic insights. pyVIPER interrogates (single-cell) RNA-seq datasets under the lens of gene regulatory networks, allowing data analysis from the perspective of the regulatory programs that govern cellular states. It doesn’t work as a “black box”. Conversely, it generates very interpretable “white box” models that allow users to make sense of their data. Finally, the implementation of this newly optimized pipeline makes sophisticated data-driven systems biology analyses accessible to users of all backgrounds.
An isoform resolution transcriptomic atlas of colorectal cancer from long-read single-cell sequencing.
Jia Chan
Senior Research Scientist
Cancer Science Institute of Singapore
What does your poster present?
The poster presents an atlas of colon cancer generated from matched long-read and short-read single-cell RNA sequencing.
Can you tell us more about the long-read sequencing technique used?
After we extracted single cells from patient samples, during our 10x Genomics (CA, USA) library prep flow we used part of the prefragmented library to generate a long-read sequencing library for PacBio (CA, USA) RNA sequencing.
Do you have any best practice tips for using these techniques?
One of the problems for long-read sequencing historically has been the volume of read counts. But now with the improved technology from PacBio, this is not so much of an issue. Using these cutting-edge technologies can save you time and bring a lot of value to your research work.
I would highly recommend using long-read sequencing! You can uncover many new things that you would otherwise miss using short-read RNA sequencing alone.
How do you hope this poster will impact cancer research?
With combined long-read and short-read single-cell RNA sequencing, we were able to identify novel transcripts that were not previously annotated. We were also able to identify alternative splicing events that were co-occurring with each other. With this new data, we hope to be able to identify new epitopes that can be used for neoantigen cancer vaccine designs.
AI-enhanced spatial proteomics reveals B cells and FOXP3 cells interactions in HPV-positive oropharyngeal squamous cell carcinoma progression.
Sumanth Reddy
Research Fellow
Mayo Clinic (FL, USA)
What does your poster present?
Here, we investigate various protein markers and their intensity at spatial single-cell resolution, using Hyperion imaging, with the end goal of identifying image-based biomarkers that could differentiate between HPV-positive oropharyngeal squamous cell carcinomas that progress and those that don’t.
The key takeaway is that this imaging mass cytometry (IMC) data was a bit of a game changer. It gives you economical single-cell resolution protein expression information, which can be used to identify markers and trends in cancers that could be used as indicators in the future to help deliver personalized medicine to patients.
Can you tell us more about the techniques used to generate and analyze this data?
First, we collected normal biopsy HND images that we used to select certain regions of interest. These regions were then sent to the Hyperion imaging system, which combines time-of-flight cytometry with immunohistochemical staining and imaging to provide IMC data. From that data, we can identify what sort of proteins are expressed within the tissue and where.
Typically, when it comes to analyzing IMC data, there is no way to manually segment or identify the cells, you can only check the level of protein level expressed in the entire tissue. You cannot do the cell segmentation or cell phenotyping based on those marker intensities, you need the tape learning algorithms presented in this poster. The crowd-test convolution neural networks we present also allow you to automate the identification of cellular niches and other factors, which is a tedious job to do manually.
Do you have any tips for best practice when using these techniques?
When it comes to analyzing IMC data in particular, there is often a task conducted called spillover correction; this is to fix the contamination of the data caused by something from a previous run, such as a metal conjugate, still being present in the machinery during the subsequent run. If the experimentalist can take care of that contamination, it would lead to higher-quality data for analysis.
How do you hope this poster will impact cancer research?
I hope we can now identify progressor and non-progressor tumors based on image-based biomarkers from images that are routinely produced when a patient is diagnosed. If we could identify early on whether the patient is going to respond to a therapy or not, then we could prescribe a better therapeutic treatment program, which would ultimately improve outcomes.
Microbial signatures and serum markers of colon cancer in African American patients
Sofia Tortora
PhD and Dentist
SUNY Downstate (Brooklyn, USA)
What does your poster present?
Our research was focused on understanding the oral–gut axis in colon cancer in a specific health disparity population: African Americans, who face disproportionately high incidence and mortality rates. Notably, periodontitis, a type of gum disease, has been linked to elevated risks of colon cancer and mortality, with African Americans exhibiting higher prevalence rates. Our study uncovered the presence of Fusobacterium nucleatum and other periodontal pathogens within colon tumors associated with alterations in gut microbiota. Intriguingly, these pathogens exhibited co-aggregation patterns resembling biofilms typically found in the oral cavity.
What techniques did you use to generate the data for this poster?
We used 16S rRNA gene sequencing to characterize the gut microbiota as well as qPCR for specific oral pathogens. We also used a novel technique called NAPPA that was developed by Arizona State University (AZ, USA). This technique allows us to see the immunoproteomic profile of the antibody response in serum, where patients with early-stage and right-sided tumors exhibited elevated anti-F. nucleatum IgG seropositivity. We also used a multiplex ELISA along with RNA-seq and immunohistochemistry.
Do you have any tips for best practice when using some of those techniques or any pitfalls to avoid?
Try to always use fresh tissue. It’s better than stool as many papers have found that stool doesn’t really represent the gut microbiome. Make sure that your tissue collection is clean – you don’t want to find contaminating bacteria in your analyses- and ensure proper collection and preservation techniques to maintain the integrity of microbial DNA. Additionally, validate your findings using complementary techniques such as qPCR or culture-based methods to confirm results.
What do you hope the impact of this paper will be on cancer research?
As a dentist, I’m hoping this research – which has found oral pathogens in the gut – will encourage physicians in oncology settings to consider the oral health status of their patients. I would love this work to facilitate interdisciplinary collaboration between dentists, physicians, and researchers, fostering a collaborative effort aimed at optimizing cancer patient care.
Your research supports a more holistic view of cancer generation. Do you find that others resist this perspective and the idea that the oral microbiome is connected to colon health?
Definitely. There’s an ongoing debate about the connection between the oral microbiome, particularly Fusobacteria, and colon cancer. One challenge lies in the precise bacterial mechanisms underlying tumorigenesis, especially given the variability in Fusobacterium colonization observed in mouse models, and the temporality between infection and cancer initiation. We advocate for more rigorous experimental methodologies, including improved in vivo models and longitudinal studies in human cohorts including a comprehensive analysis of the oral microbiome changes in association with oral condition that might be present.
Data-driven discovery of cancer-specific targets for hepatocellular carcinoma using spatial transcriptomics
Hongyoon Choi
Chief Technical Officer
Portrai, Inc. (Seoul, South Korea)
What does your poster present?
This poster presents a novel approach to target discovery, integrating spatial transcriptomics and single-cell RNA-sequencing data to advance cancer therapeutics. Targeted drug delivery modalities like antibody–drug conjugates (ADC) and radioligand therapy (RLT) have changed many oncology fields. These platforms target tumor-specific molecules, delivering therapeutic agents or radioisotopes systemically. Ideal targets for ADC and RLT are characterized by conditions such as specificity to cancer cells, expression on the cell surface, and their absence in normal cells of critical organs like the lung, liver or kidney.
To identify such targets, we have developed a data-driven discovery process that utilizes comprehensive gene expression data at the cellular level obtained using spatial transcriptomics. At AACR 2024, we showed its application in studying hepatocellular carcinoma. By refining target selection, this approach aims to improve the precision and effectiveness of emerging cancer treatments.
What techniques did you use to generate the data for this poster?
In this study, we employed spatial transcriptomics. This method offers comprehensive gene expression mapping with near-cellular resolution in a single experiment. Tumors exhibit heterogeneity; they comprise diverse cell types, each with unique biological functions and spatial interactions within the tumor microenvironment. Consequently, understanding these cell types and their interactions is crucial for unraveling tumor biology.
Do you have any best practice tips for using these techniques?
The key to effectively utilizing spatial transcriptomics lies in clearly defining the research questions you aim to answer. For our case, we hypothesized that spatial transcriptomics could revolutionize target discovery by enabling the visualization of all potential molecular targets within their spatial context in actual tissue samples. Therefore, we thought that a new algorithm could have the potential to connect this extensive data platform with cutting-edge cancer treatments. Understanding the specific biological or clinical questions at the outset will guide the experimental design, data collection and analysis for spatial transcriptomics.
How do you hope this poster will impact cancer research?
Targeted drug delivery platforms like ADC and RLT have received approval for some solid tumors, significantly altering cancer therapy paradigms. Yet, the expansion of their applications across diverse cancer types hinges on identifying new and optimized targets, which currently presents a challenge. In this context, this extensive data provided by spatial transcriptomics, capturing the complex biology of heterogeneous tumors, can address this critical need. We are confident that the exponentially growing data from spatial transcriptomics will enhance ADC and RLT strategies through our algorithm- and data-driven techniques.
The opinions expressed in this interview are those of the interviewee and do not necessarily reflect the views of BioTechniques or Taylor & Francis Group.