AI for an eye: AI speeds up retinal imaging
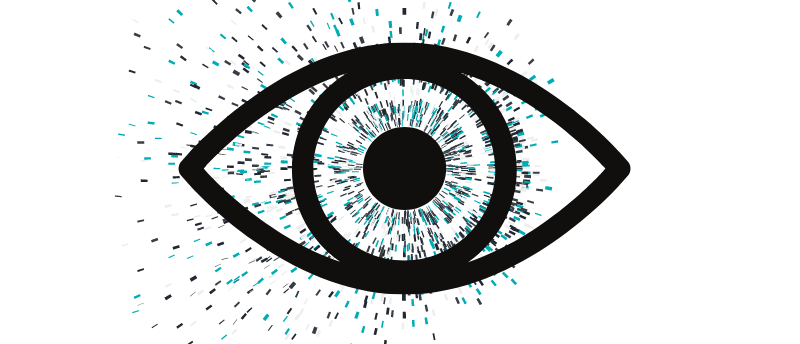
Researchers have developed an AI tool that makes retinal imaging 100 times faster.
Retinal diseases are one of the leading causes of blindness; however, imaging cells in the retina in detail can be challenging and time-consuming. Now, researchers from the National Institutes of Health (NIH; MD, USA) have developed an AI tool that can be integrated into a common retinal imaging technique to produce high-resolution images in a shorter amount of time.
If you’ve had an eye test in the past few years, then you may have been offered an optical coherence tomography (OCT) scan. OCT is a noninvasive imaging technique that takes 3D images of eyes by sending a beam of light into the tissue and measuring the reflected light. By analyzing the time delay and intensity of the reflected light, OCT can generate detailed images of the internal structures of tissues.
To improve OCT’s lateral resolution, OCT has been combined with adaptive optics (AO), a technology initially developed in astronomy, which reduces optical aberrations, resulting in sharper and clearer images. AO-OCT can visualize 3D retinal structures at cellular-scale resolution, including the retinal pigment epithelium (RPE), a layer of tissue behind the light-sensing retina that is implicated in many diseases of the retina.
“Adaptive optics takes OCT-based imaging to the next level,” said corresponding author Johnny Tam. “It’s like moving from a balcony seat to a front-row seat to image the retina. With AO, we can reveal 3D retinal structures at cellular-scale resolution, enabling us to zoom in on very early signs of disease.”
More than meets the eye: how molecular aging can give an insight into health
Eyes are not only the window to the soul but also to age and health status.
However, AO-OCT comes with its challenges, such as speckle, which is noise inherent to the imaging process. To overcome this, researchers repeatedly image cells over an extended period. As time progresses, the speckle shifts, revealing different parts of the cells that were previously obscured. These images are then pieced together to create a speckle-free image, but this is a laborious and time-consuming task.
To cut down the time taken to acquire speckle-free AO-OCT images, Tam and his team developed an AI tool called parallel discriminator generative adverbial network (P-GAN) to recover speckle-obscured cellular features from a single AO-OCT volume, circumventing the need for acquiring a large number of volumes for averaging.
They trained P-GAN using nearly 6000 manually analyzed AO-OCT-acquired images of human RPE, each paired with its corresponding speckled original.
The team tested P-GAN on new images and found that it successfully removed speckle, uncovering cellular details. With just one image capture, P-GAN produced results comparable to the manual method, which required acquiring and averaging 120 images. The team estimates that P-GAN reduced imaging acquisition and processing time about 100-fold and improved image contrast 3.5-fold.
“Our results suggest that AI can fundamentally change how images are captured,” commented Tam. “Our P-GAN artificial intelligence will make AO imaging more accessible for routine clinical applications and for studies aimed at understanding the structure, function, and pathophysiology of blinding retinal diseases. Thinking about AI as a part of the overall imaging system, as opposed to a tool that is only applied after images have been captured, is a paradigm shift for the field of AI.”