Doubling up: novel AI models improve de novo peptide sequencing
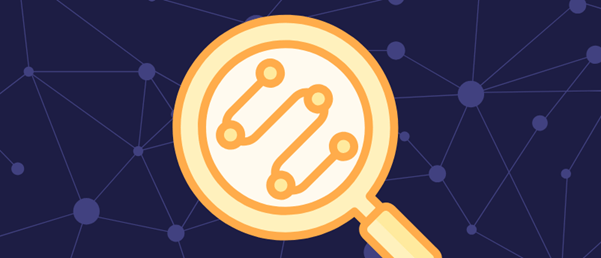
Two new AI models work together to enable more precise de novo peptide sequencing.
Researchers from the Technical University of Denmark (DTU; Kongens Lyngby, Denmark), Delft University of Technology (Netherlands) and AI company InstaDeep (Cambridge, UK) have developed two novel AI models that can more precisely identify peptides from mass spectrometry data.
Mass spectrometry-based proteomics identifies the peptide that generates a tandem mass spectrum. Traditional methods rely on matching the unknown peptide with a known one in a protein database; however, databases can be limited, and deep searches are time-consuming and demand high computing power. This approach also fails to recognize novel peptides.
De novo peptide sequencing, on the other hand, assigns peptide sequences to spectra without prior information. While de novo sequencing can identify novel peptides, the performance of current algorithms compared to database searches remains underwhelming.
Updates from the experts: bioimage analysis, genomics in space, imaging cytometry and epigenomics
Learn about experts’ favorite pieces of tech and key takeaways from ABRF 2025 (23-26 March; Las Vegas, NV, USA).
To address this, the team developed two new AI models, InstaNovo and InstaNovo+. InstaNovo is a transformer model that interprets mass spectra by mapping fragment ion peaks to peptide sequences, predicting the next amino acid in a peptide sequence. InstaNovo+, a diffusion-based iterative refinement model, can then be used to refine these predictions by mimicking how researchers manually refine peptide predictions.
“Seen together, our models exceed state-of-the-art and are significantly more precise than currently available tools,” commented co-first author Kevin Michael Eloff (InstaDeep).
The researchers conducted various experiments to assess the real-world application of the models. For example, they applied the models to the sequencing of human leukocyte antigens and found that the models identified thousands of new peptides that were not found using traditional methods. The newly discovered peptides could be potential targets in personalized cancer treatments.
The researchers believe that the success of the models so far has implications extending beyond medical sciences. “Looking at it from a purely technical, scientific perspective, it is also true that with these tools, we can improve our understanding of the biological world as a whole, not only in terms of healthcare but also in industry and academia,” explained corresponding author Timothy Patrick Jenkins (DTU). “Within every field using proteomics – be it plant science, veterinary science, industrial biotech, environmental monitoring or archaeology – we can gain insights into protein landscapes that have been inaccessible until now.”