Integrative omics for characterizing addiction and brain development
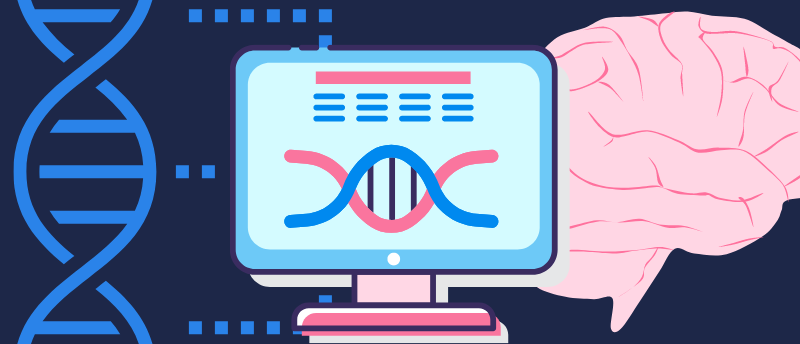
Melyssa Minto (left) is an omics researcher and bioinformatics scientist at RTI International (NC, USA), where she uses multiomic data to understand how the central dogma of biology – the way genetic information flows from DNA, to RNA, to protein, or alternatively, from RNA directly to protein – relates to a phenotype or a disease.
In grad school, Minto’s focus was on the neurogenomics of addiction and development. She used different omics data – whether that be chromatin data, gene expression data or specific protein-binding data – from various parts of the mouse brain to understand how these pieces play together in paradigms of addiction or throughout development.
In this interview, Digital Editor Beatrice Bowlby spoke to Minto about her research, the challenges of integrating complex, multi-layered data, the future of multiomics and what it means to be a multiomic bioinformatician.
What has your research characterizing gene networks illuminated about substance use disorders and neurobiology more broadly?
Neurons are very long-lived cells. Mature neurons don’t divide; they don’t make more of themselves. Therefore, sometimes the way that they change their function in response to stimuli requires first activating networks of gene expression. I wanted to know how these epigenomic driven transcriptional changes were happening: which signals are activating which genes? Where is RNA machinery binding to activate those genes? Plus, what kind of proteins are binding to DNA and signaling to other molecules to modify the genome?
For example, in one of my studies in grad school, we were looking at amphetamine addiction in mice, and we knew about this important area in the nucleus accumbens that modulates addictive behaviors. So, we wanted to know how the cells in that area were being activated to modulate addiction. We were asking questions like where are the proteins binding? Which genes are being turned on and which are being turned off?
We expected to find that when we stimulate a cell, the DNA becomes more open such that RNA polymerase can bind, and certain genes are then turned on. However, that is not what we found! We found that the regulatory regions of the genes were already in a fixed chromatin state. Because gene expression changes were not preceded by changes in the chromatin state, it was clear that it was the availability of proteins that bind to those regions to modulate expression that changed. It was interesting to find that the chromatin state of our neurons may be more static, whereas the genes that were being turned on and turned off is a little bit more dynamic.
How can our understanding of epigenomics be enhanced with computational tools?
There are so many different layers of epigenomics. The first epigenomic marker I learned about was DNA methylation, which is a small methyl mark on a nucleotide, and when methylation accumulates it can make chromatin more condensed. This condensed chromatin makes it harder for proteins to bind, meaning that genes in those areas of chromatin are less likely to be turned on. Additionally, several epigenomic modifications can be made to the histone tail; the tail can be methylated, acylated, phosphorylated, etc., and each of these modifications has a different role or targets different regions of the genome. So, there are a lot of epigenomic marks out there, and I think with good computational tools we can do different kinds of analyses on epigenomic modification data to better understand what those marks are for, where we see those marks and how they are relevant to human disease.
One can use many different analysis techniques for investigating epigenomic modifications. One type of analysis we use is a factor analysis, which reduces the dimensionality of a matrix composed of marks and their associated gene expression. This sort of analysis helps us better understand how epigenomic marks relate to a phenotype or disease, providing a more comprehensive snapshot of this relationship than we could capture without computational tools.
What statistical techniques and bioinformatics tools do you use – or have you developed – to integrate and analyze genomic data?
The first part of every analysis is about understanding your data. All omics data goes through a pre-processing quality control step and then some kind of differential testing; for example, for gene expression data you do differential expression analysis, and for chromatin immunoprecipitation sequencing (ChIP-Seq) data – where you are getting protein-binding locations – you can do differential binding analysis. The next step is layering your multiomic data. When you have multiple omics from the same cells or the same disease, you can layer that data on each other to get more out of the data.
One study I was a part of was looking at a specific transcription factor, Zic, that has been associated with the development of the cerebellum. My question was how is Zic orchestrating the right set of genes to be turned on and turned off for the cerebellar cells to be at the right place at the right time and mature in the right way? I was lucky to have gene expression data and Zic’s ChIP data, so I knew where Zic was binding and at what relative quantities. We also leveraged chromatin confirmation data that had recently come out from another lab, which basically told us what parts of the genome were close to each other in proximity. This gave us information about long-range interactions, and what I was able to do was use these interactions to tie enhancer binding of Zic to its appropriate gene. A more naive approach might be to just assign a binding event to the nearest gene, but that may not be where it is actually affecting. Therefore, overlaying the chromatin confirmation data on top of the gene expression data allowed me to more appropriately assign what genes Zic was binding to and regulating, revealing whether Zic binding was associated with upregulation of a gene or downregulation of a gene.
Combining all that data provided us with a clear picture of how Zic was behaving. At the time, the science was saying that Zic is important in one stage of development, but we saw that Zic is actually important at multiple stages of development: first, regulating the genes that are important in the migration of these cells, and second, supporting the development of the synapses of these cells. Using all this data, I developed an analysis to determine the molecular mechanisms of how this one transcription factor regulates essentially the whole maturation of cerebellar cells.
What are the biggest challenges that you face when doing integrative omics analyses and how do you currently tackle these challenges?
One of the greatest challenges in integrating data is that you really must be sure about your assumptions. There are a lot of assumptions that are made when developing integrative tools like this. Because of this, I think it’s really important to have other bioinformatics scientists around to discuss your analysis plan. The assumptions must make sense from a computational standpoint, from a statistical standpoint and from a biological standpoint. They all have to be in agreement, and that is a huge challenge.
In terms of integrating the data from a computational perspective, there were some Zic peaks that did not overlap with any of the chromatin confirmation loops. So, we didn’t get to use 100% of the data, but, hopefully, what we are doing when we layer so much data on top of each other is increasing the confidence and providing a more detailed picture of the transcription factor’s role in regulating development.
Another challenge is keeping track of the lineage of your data. When you are working with data and doing all these different manipulations, it is really important to have all that very well documented, saved and backed up. That way you can trace your steps back; if you don’t save your data along the way, then you will get to the end and wonder how exactly you got there. You’ll be asking yourself what version of the data you’re using. So, data provenance is massively important!
What role do you think multiomic analyses and bioinformatics tools will play in life science research in the future?
I think it’s going to become standard to do multiomic analyses. It’s just so important to have the full picture of the central dogma because we can have genes that upregulate or downregulate, but what does that mean on the protein level? Are we actually seeing an increase in protein? And are those proteins doing what we think they are doing?
Additionally, when we think about human disease, there are a lot of comorbidities. Speaking from the substance use disorder space or the psychiatric disorder space, there are a lot of different diseases within that space that have shared gene associations. We see that some genes that are important in schizophrenia are also important in bipolar disorder. So, realistically, we can’t look at these genes that are involved in two different conditions and say that they’re completely separate. In the future, we may not be doing multiomics in the sense of different omics within the same disease, but maybe we’ll be investigating a single omic across different diseases to then characterize how those diseases interact with each other and how one might affect the other. I definitely think multiomics and associated analyses will be the standard in terms of understanding human diseases.
The opinions expressed in this interview are those of the interviewee and do not necessarily reflect the views of BioTechniques or Taylor & Francis Group.