Machine learning approaches for spatial omic data analysis
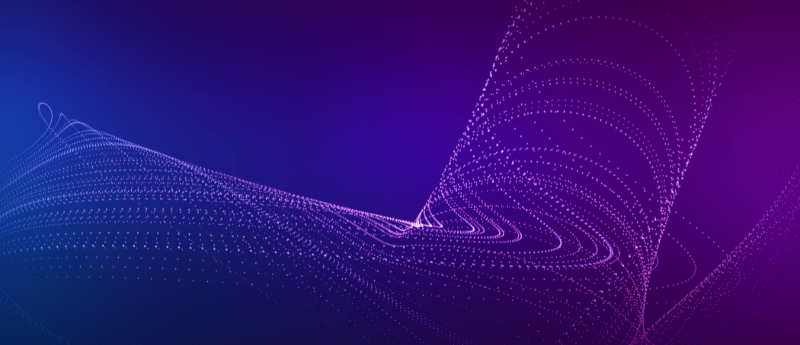
Jeremy Goecks (left) is the Assistant Center Director for Research Informatics at the Moffitt Cancer Center (FL, USA), where he is also an Associate Faculty Member in the Department of Machine Learning. Jeremy’s computational research lab leads the development of machine learning-based models for the analysis of spatial omic data.
We caught up with Jeremy at AACR 2024 to discuss his lab’s work, get best practice tips for collecting and analyzing spatial omic data and discuss how the field can progress in the future.
What did you present at AACR?
I had two stories that I shared with the audience. The first covered our recent publication detailing the use of spatial omics and machine learning to understand how a novel immunotherapy, agonistic CD40, impacted the tumor microenvironment in pancreatic cancer. We also used these approaches to explore how well we could predict which individuals would respond to that immunotherapy and have long disease-free survival and vice versa. The second story is about how we can develop software to make it easier to run these types of complex analyses, whether you’re an experimental or computational investigator.
What were three key takeaways from your presentation?
Number one is that team science is really important. The publication that I shared with the audience was a fantastic collaboration between myself and several immunologists at Oregon Health & Science University including Katelyn Byrne and Lisa Coussens. And the combination of my data science expertise and their expertise in immunology was really important in making the project a success.
Takeaway number two is that there are so many different things that you can measure from a single-cell perspective and from a spatial perspective in these tumors that it’s hard sometimes to know which features are important. Machine learning is one way that you can get that information and identify which features are helping us understand what’s happening with a tumor’s biology.
My lab has demonstrated that machine learning can be used to identify which features of the many thousands that you can measure are important, both for understanding how the therapy is modifying the tumor microenvironment and what features are important for predicting response.
The third is that these data science experiments are hard to run and take a long time right now. We need to build better software to accelerate that process, reduce the amount of time it takes to run these analyses while ensuring that they’re robust, reproducible and useful to the scientific community.
Single-cell spatial proteomics
This In Focus explores how spatial proteomics has granted researchers access to information regarding the cell-surface proteome, providing examples of their applications across the life sciences.
What are your best practice tips for getting the most out of that spatial omics data?
One of the first things you need to do is harmonize all the data and metadata available. You want to create a dataset where all the data has been processed in the same way and it has the necessary associated metadata, so you know if you’re missing anything and exactly where each data point comes from, including which patients and at which time points.
From a data science perspective, best practices focus on using reproducible approaches; whether that’s GitHub to store your code or using automated pipelines to run your analyses and produce your figures. This means when the time inevitably comes to reproduce that analysis or apply it to a new data set, you’re able to do so confident that you are repeating the analysis exactly.
What exciting contributions has your lab made to spatial omic data analysis that your lab has delivered?
We are trying to use machine learning to improve both our ability to predict a therapeutic response and our understanding of the underlying biology that leads to said response. We can interrogate our models to find out why they made a specific prediction and identify which biological features they are using. This avoids the typical “black box” issue of a lot of AI models, where they answer a question but can’t explain how they arrived at the answer.
The other major contribution, I would say, is trying to keep the models as simple as possible. We use relatively simple machine learning models but are still able to demonstrate good performance on our data sets. You don’t always need the most complex algorithms in order to get the signal out of the data if the data set is strong.
What can researchers do at the data generation stage to help improve their downstream analysis?
I always encourage my experimental colleagues to make sure that they record all the elements of their experiment. Everything from the antibodies and sample IDs that they used to the particular microscopy focal levels used. All the metadata that they have available to them should, to the best of their ability, be recorded in a systematic way.
The second thing I think is relatively straightforward, but it’s to try to process all your samples in the same way. If you process your samples in the same way, it’s much easier to computationally analyze them and not worry that there are technical differences that are coming out in the analysis rather than the biological differences that you really want.
If there was one thing you could ask for to maximize the impact of spatial omics data in cancer, what would it be?
If there was one thing, I would say sharing more of these datasets widely from the raw images to the downstream single-cell omics tables would really be at the top of my list. Making this data open would allow lots of other analysts to come and put their hands on it, to connect it with other data sets, and these larger data sets ultimately, I think, is how we will accelerate cancer research and improve patient care as a community.
The opinions expressed in this interview are those of the interviewee and do not necessarily reflect the views of BioTechniques or Taylor & Francis Group.