Machine learning helps distinguish between rheumatoid arthritis subtypes
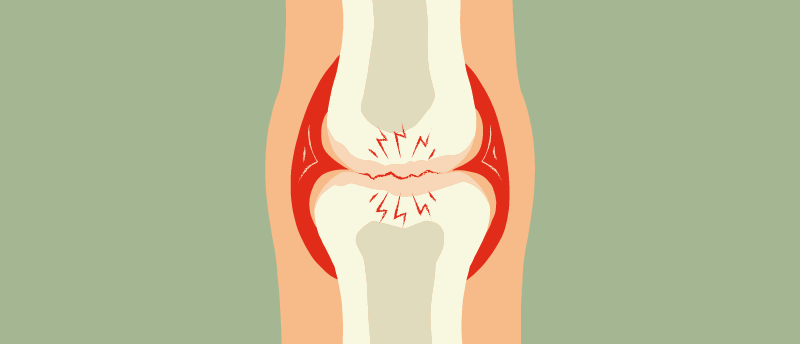
Researchers have developed a machine-learning tool that can subtype samples from people with rheumatoid arthritis.
A collaboration between Weill Cornell Medicine (NY, USA) and the Hospital for Special Surgery (NY, USA) has developed a machine-learning tool that can distinguish between three subtypes of rheumatoid arthritis. The tool is quicker and more cost-effective than traditional methods of subtyping and can be used to help guide treatment decisions.
Rheumatoid arthritis (RA) is an autoimmune-mediated inflammatory disorder that primarily affects joints. Recent advances in histopathological studies of RA synovial tissue heterogeneity revealed three distinct types of RA based on cellular composition. Determining which RA subtype a patient has can help guide more effective treatment strategies. However, classifying RA subtypes is currently a manual process, which is time-consuming and may lead to inconsistencies between pathologists.
To address this, the team developed an automated multi-scale computational pathotyping (AMSCP) machine-learning model for the automatic analysis of tissue samples, similar to those seen in oncology.
The team trained the algorithm on RA samples from two sets of mouse models. The first was used to optimize the model’s ability to distinguish tissue and cell types in the sample and sort them by subtype, and the second set was used to validate the tool. The tool has two distinct components: it can segment different tissue types to characterize tissue-level changes, and it can classify cell types within each tissue compartment to study how these change across disease states.
What’s happening in the world of bioengineering?
Here, we outline the recent bioengineering feats that have contributed to our understanding of the human body as well as the importance of safe data-centric research.
The tool successfully classified the RA subtypes in the mice and even showed that cartilage degradation was reduced within 6 weeks of administering commonly used RA treatments, suggesting that the tool could provide new insights into the disease that humans might miss.
The team then tested the tool on human samples from the Accelerating Medicines Partnership Rheumatoid Arthritis research consortium and found that the tool effectively and efficiently typed human clinical samples of RA. The team is now further validating the tool with more human samples and determining how to incorporate it into pathologists’ workflows.
“It’s the first step towards more personalized RA care,” commented corresponding author Richard Bell. “If you can build an algorithm that identifies a patient’s subtype, you’ll be able to get patients the treatments they need more quickly.”
The researchers are now working on developing similar tools for other diseases. Co-author Fei Wang’s team has already demonstrated that machine learning can distinguish three subtypes of Parkinson’s disease. “We hope our research will trigger more computational research on developing machine learning tools for more diseases,” concluded Wang.