You gotta know how to fold ‘em: new computational model to predict protein folding
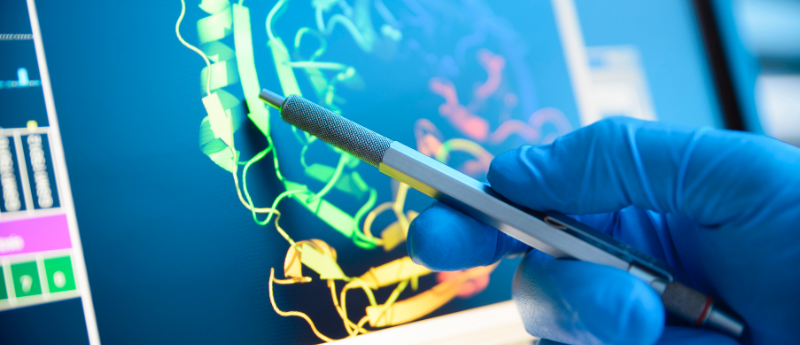
A new statistical model demonstrates the ability to correctly predict the folding mechanisms for both small and large proteins.
The function of a protein is determined by its structure. From the rigid fibers of keratin to the catalytic active sites of digestive enzymes, a series of complex chemical interactions shape a protein into its functional form. Given the sheer complexity of proteins as molecules, thousands of amino acids strung together in a polypeptide chain, contorted and manipulated by interactions between amino acids, post-translational modifications and co-ordinations, determining a protein’s structure can be an immensely difficult endeavor, often taking years of dedicated research.
Understanding the proper structure and function of proteins is vital to further our knowledge of disease pathologies and to find targets for novel therapeutics. Computational methods have increasingly been employed to expedite the prediction of protein structure. Most recently, DeepMind’s AlphaFold2 utilized AI deep learning to predict protein structure from a base amino acid chain. However, predicting how a protein achieves this structure in nature is currently outside the capabilities of AlphaFold.
In a recent development, researchers from the University of Tokyo (Japan) have developed a computational system that exploits statistical mechanics to not only predict the final structure of the protein but also the stages it takes to form this structure. Their model successfully predicted the folding of both small and large proteins, concordant with experimental results.
The technique is an expansion of the existing Wako-Saitô-Muñez-Eaton (WSME) statistical model. The WSME model can map the folding pathways of small single-domain proteins. Multidomain proteins, which make up most of the human proteome, remain outside the capabilities of the WSME model.
AlphaFold computational modeling in drug discovery: the good, the bad and the AI
A new study has utilized AlphaFold machine-learning software to find potential targets in the Escherichia coli (E. coli) essential proteome for developing novel antibacterial drugs.
The new model, WSME-Linker (or WSMEL) developed by Koji Ooka and Munehito Arai differs from the WSME model in one crucial way; allowing nonlocal interactions between distant amino acid residues to be considered. This is done by introducing virtual linkers into the model, which act as surrogates for these nonlocal interactions. Going further, the model can also incorporate disulfide bridges, a covalent interaction between two cystine amino-acid residues, vital to the final structure of many proteins, particularly those that exist completely or partially outside of cells.
“Our theory allows us to draw a kind of map of protein folding pathways in a relatively short time; mere seconds on a desktop computer for short proteins, and about an hour for large proteins, assuming the native protein structure is available by experiments or AlphaFold 2 prediction,” said co-author Arai, describing the key achievements of the study. “The resulting landscape allows a comprehensive understanding of multiple potential folding pathways a long protein might take.”
The potential of this model for the biomedical research world is potentially limitless. There are around 20,000 proteins in the human proteome, yet only around 100 have had their folding mechanisms studied in detail. This development has the potential to shed light on a number of diseases, including Alzheimer’s and Parkinson’s, both of which are closely associated with the incorrect folding of proteins.
Moreover, Arai is hopeful that the new model ‘may be useful for designing novel proteins and enzymes which can efficiently fold into stable functional structures, for medical and industrial use,’ meaning this new model could not only open doors for drug discovery in the future but also expand the horizons of biocatalytic research.