When can we trust AI?
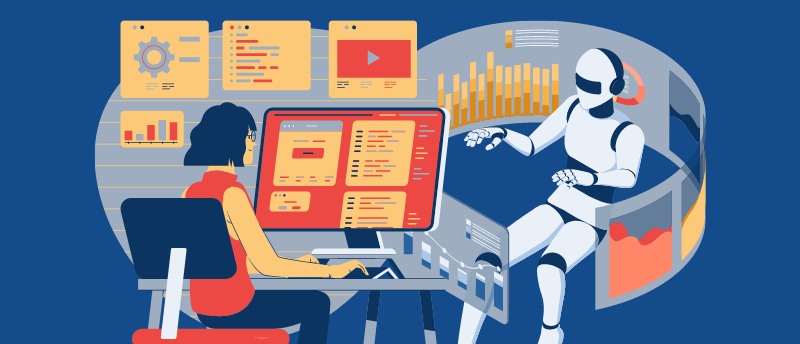
AI models aren’t infallible; that’s why a prediction is often accompanied by a confidence score. Thanks to a recent study, these uncertainty estimates are now more accurate, efficient and scalable.
Researchers at Massachusetts Institute of Technology (MIT; MA, USA) have developed a technique – called IF-COMP – to improve the accuracy and efficiency of these uncertainty estimates in machine-learning models, improving their utility for researchers and clinicians.
Machine learning is becoming more and more commonplace in the life sciences, from predicting certain behaviors in animals to analyzing medical images to identify diseases. For such scientific and medical purposes, it is essential that users know the uncertainty estimate of a given output or prediction.
If a model identifies a pleural effusion in a medical image with 49% confidence, then we expect the model to be correct 49% of the time; however, these estimates are only useful when they’re accurate. Not only is there a push to make these uncertainty estimates more accurate and efficient, but it is also important that they are applicable to large deep-learning models being used in safety-critical situations. Not all end users are machine-learning experts and therefore the better the information output, the more informed decisions can be made.
“It is easy to see these models perform really well in scenarios where they are very good, and then assume they will be just as good in other scenarios. This makes it especially important to push this kind of work that seeks to better calibrate the uncertainty of these models to make sure they align with human notions of uncertainty,” commented lead author Nathan Ng.
The traditional uncertainty quantification methods require complex calculations, which can make scaling to the millions of parameters within machine-learning models difficult. There is also the added complexity of these methods requiring users to make assumptions about the data used to train the model, as well as the model itself.
To avoid the need for users to make assumptions about the model used, the research team utilized the minimum description length principle (MDL), which is used to better quantify and calibrate uncertainty in test iterations of the model.
Can AI predict the development of autoimmune diseases?
A new study details how an AI algorithm has been trained to identify genes associated with autoimmune diseases, hopefully allowing for earlier intervention.
The MDL considers all potential labels a model could assign to a given test point, the uncertainty estimate is then calculated based on the number of alternative labels – the ones not chosen by the model – that also fit the test point well, decreasing the model’s confidence. Each data point is labeled with a certain amount of code, known as stochastic data complexity; when the model is confident in a prediction, the code is short, but with increasing uncertainty, the code length increases. However, the MDL would be very slow and require a great deal of computational power if it had to assess all potential labels for each data point.
Therefore, the team developed IF-COMP to make MDL quick enough for use with large deep-learning models deployed in healthcare settings. IF-COMP is an accurate approximation technique that can estimate stochastic data complexity using an influence function. Alongside this function, the team utilized a statistical technique, temperature scaling, that improves the model’s output calibration. Together, the influence functions and scaling can accurately approximate the stochastic data complexity, resulting in the efficient production of uncertainty quantifications reflecting a model’s true confidence.
When tested, IF-COMP demonstrated faster, more accurate uncertainty quantification than other methods. It can also be applied to many different machine-learning models, making it more versatile. In future, the researchers want to apply IF-COMP to large language models as well as investigate other uses for the MDL.