Who won the 2024 Nobel Prize in Physics?
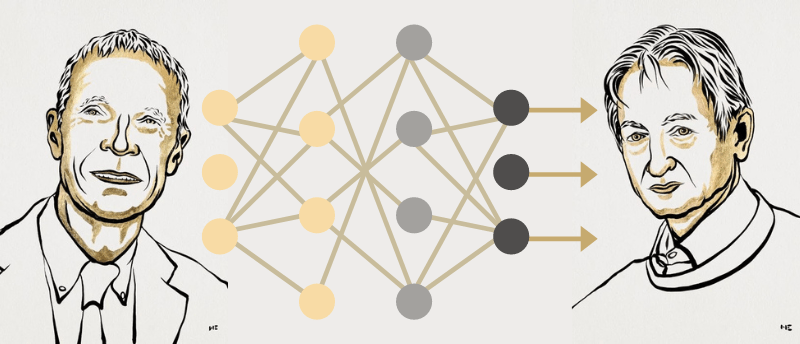
John Hopfield and Geoffrey Hinton have won the 2024 Nobel Prize in Physics “for foundational discoveries and inventions that enable machine learning with artificial neural networks.”
This year, the Nobel Prize has recognized John Hopfield (Princeton University, NJ, USA) and Geoffrey Hinton (University of Toronto, Canada) for their use of physics tools to help lay the foundation for today’s powerful machine learning systems [1].
Artificial neural networks
Machine learning has been in development for decades, only sparking recent widespread interest in the last few years. However, this artificial intelligence has been of interest to researchers for many years as, it can be used to sort and analyze vast amounts of data. When we discuss machine learning and artificial intelligence, we are usually talking about the artificial neural network structures underlying these computational systems. These networks are called artificial neural networks because they mimic brain functions such as memory and learning.
Comparing natural and artificial neurons. ©Johan Jarnestad/The Royal Swedish Academy of Sciences [2].
Artificial neural networks are composed of nodes, representatives of neurons, that have different values. These nodes are connected and pass information between each other in a similar fashion to the information exchange that takes place at a synapse between neurons. These nodes can be made stronger or weaker depending on the amount and frequency of information transferred to them during the training the networks undergo.
The Hopfield network
In 1982, John Hopfield invented an associative memory network that can save and recreate patterns in data, such as images. The Hopfield network utilizes a physics principle that describes a material’s characteristics based on its atomic spin, a property that makes each atom a tiny magnet. The spins of neighboring atoms affect each other and the energy levels in a system.
The Hopfield network is built on this idea of nodes having a certain energy and is trained to make connections between nodes to result in saved patterns having low energy. This way, when the network is fed an incomplete pattern, it works through each node and updates their values to ensure the network’s energy lowers, taking a step-by-step approach to identify the stored pattern that is most like the incomplete or distorted pattern the network was fed.
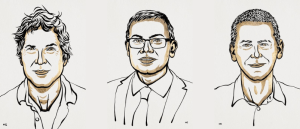
© Nobel Prize Outreach.
Who won the 2024 Nobel Prize in Chemistry?
This year, the 2024 Nobel Prize in Chemistry has been awarded in half to David Baker for computational protein design and half jointly to Demis Hassabis and John Jumper for their creation of an AI model that can predict protein structure.
The Boltzmann machine
Geoffrey Hinton used the Hopfield method and statistical physics to develop a new network called the Boltzmann machine, which can learn to autonomously recognize specific elements in a data type. Statistical physics is used to analyze the states in which individual components can jointly exist, such as gas molecules. Depending on the amount of available energy, some states are more probable than others – a concept described by an equation from physicist Ludwig Boltzmann.
This network is composed of two node types: visible and hidden nodes. The visible nodes intake and output information while the hidden nodes are essential to network function. The network is trained on examples likely to arise when the machine is run, and each node is fed a rule for updating its value, facilitating pattern change amongst the nodes in the network without changing the network’s properties. Each pattern is assigned a specific probability depending on the network configuration’s energy according to the Boltzmann equation. Therefore, this network can classify images or create examples of the type of pattern that was used to train it.
Past and present perspectives
Since the 1980s, John Hopfield and Geoffrey Hinton have been laying the foundation of modern machine learning utilizing physics principles. Today, the vast amount of data available for training networks and the massive increase in computing power has propelled machine learning forward and led to the advent of deep learning models consisting of multiple layers of nodes and connections.
Machine learning’s applicability to scientific analyses has made it an attractive tool among many life science fields, being utilized for predicting molecular and material properties. Now, researchers are expanding machine learning’s applications, raising ethical questions regarding the use of artificial intelligence and presenting interesting possibilities for the future of its use.