All in a drop’s work: laying the groundwork for infrared molecular fingerprinting
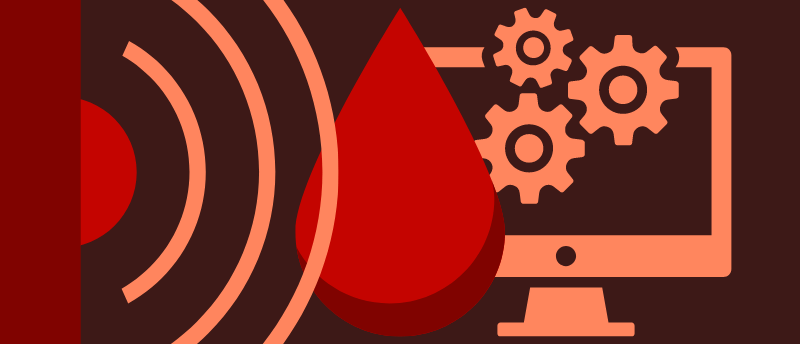
A new study has set out to transform molecular diagnostics, utilizing infrared light and machine learning to detect health conditions from a single drop of blood.
Researchers from the Broadband Infrared Diagnostics (BIRD) team at Ludwig-Maximilians-Universität München (LMU) and the Max Planck Institute of Quantum Optics (MPQ; both Munich, Germany), led by Mihaela Žigman, have developed a method for effectively and rapidly screening human health. Utilzing infrared spectroscopy and machine learning, the method lays the groundwork for more efficient detection and management of conditions, especially metabolic disorders.
Infrared spectroscopy has been a key tool in chemistry for decades; however, it has yet to become an established diagnostic technique. It’s a physio-chemical technique that uses infrared light to analyze the molecular composition of substances, giving each molecule a fingerprint. Its ability to reveal detailed profiles about molecular signals means it has great potential as a medical diagnostic tool. The current study demonstrated why, hoping to integrate it into medical practice.
The BIRD group sought out a collaboration with Annette Peters’ team at Helmholtz Zentrum München (Germany) to develop infrared molecular fingerprinting based on blood samples from thousands of individuals in the KORA study, which is a comprehensive health research project based out of Augsburg, Germany. They analyzed 5,184 blood samples from 3,169 individuals; samples were collected between 2006 and 2008 and then again between 2013 and 2014.
Talking Techniques | Cytokines: from therapeutics to diagnostics
In this episode of Talking Techniques, Ritwika Biswas, Field Application Scientist at Sino Biological US Inc. discusses the use of cytokines in immunotherapy. Ritwika details the role of cytokines in the body, before going on to discuss how they can be used as therapeutics and to guide treatment decisions. Ritwika also shares how she thinks these proteins will be used in the future.
After using infrared spectroscopy to obtain molecular fingerprints from blood samples, the team analyzed the fingerprints using machine learning, correlating them with medical data. They found that the fingerprints did indeed contain important information that could enable rapid health screening; the algorithm was able to detect and distinguish between various health states, such as abnormal levels of blood lipids, changes in blood pressure and type-2 diabetes.
The algorithm could also identify healthy individuals that remained healthy throughout the course of the investigation. Not only could the method identify healthy individuals and singular conditions, but it could also detect complex conditions involving multiple illnesses simultaneously. What’s more, it can predict the development of metabolic syndromes many years before symptoms appear.
Utilizing this infrared molecular fingerprinting to monitor metabolic disorders would allow for earlier detection and therefore earlier intervention, almost certainly improving outcomes. The researchers are hopeful that as technology advances and this method becomes more common, there may be a greater push toward personal health monitoring, which would allow individuals more autonomy over their health.
This study highlights that the combination of infrared spectroscopy and machine learning offers a promising medical diagnostic tool, requiring only a single drop of blood to transform health profiling and monitoring.