Automated lung disease diagnosis
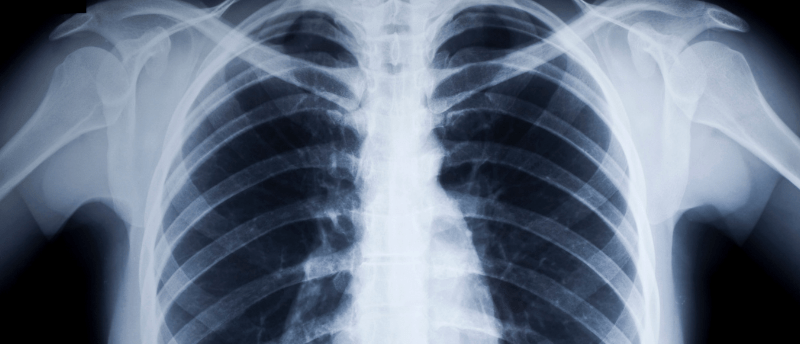
Artificial intelligence (AI) used to diagnose lung diseases may reduce winter hospital waiting times.
Researchers at the University of the West of Scotland (UWS; Paisley, UK) have developed an algorithm that can diagnose lung diseases, including tuberculosis and pneumonia, from X-rays with 98% accuracy. The use of AI for automatic diagnosis of lung diseases holds the potential to reduce waiting times in hospitals and relieve the strain on already burdened hospitals.
Infectious diseases affecting the lungs can be particularly hazardous during the winter period. Diagnosing these diseases is typically expensive and time-consuming as multiple tests, including CT scans, blood tests, X-rays and ultrasounds, are usually required. This often leads to long waiting times, which will likely be intensified following the COVID-19 pandemic.
Naeem Ramzan, Director of the Affective and Human Computing for SMART Environments Research Center at UWS and lead researcher on this project, commented: “There is no doubt that hospital departments across the globe are under pressure and the outbreak of COVID-19 exacerbated this, adding further strain to pressured departments and staff. There is a real need for technology that can help ease some of these pressures and detect a range of different diseases quickly and accurately, helping free up valuable staff time.”
One scan is all it takes: diagnosing Alzheimer’s disease
Researchers have trained a machine-learning algorithm to recognize signs of Alzheimer’s disease in magnetic resonance imaging of the brain.
Cutting-edge AI technology developed during the pandemic by UWS, which was used to detect COVID-19 from X-ray images, has now been further advanced to recognize multiple lung diseases within minutes. The research team developed an algorithm that analyzes patients’ X-ray images and compares them to a database of thousands of images from patients with pneumonia, tuberculosis and COVID-19. This machine-learning platform uses a deep convolutional neural network algorithm, which is typically used for analyzing visual imagery, to recognize and identify diseases with 98% accuracy.
Milan Radosavljevic, Vice-Principal of Research, Innovation and Engagement at UWS, noted: “I am excited about the potential of this innovative technology, which could help streamline diagnostic processes and reduce strain on staff. It’s another example of purposeful, impactful research at UWS, as we strive to find solutions to global challenges.”
If implemented as standard procedure, the researchers believe that this highly efficient process will relieve the pressure on radiographers, reduce waiting times and reduce costs by cutting out unnecessary diagnostic tests. The team at UWS is now looking to apply the algorithm to other diseases for which X-ray images are utilized, including cancer.