Designing new tools for multiomic explorations into the human genome
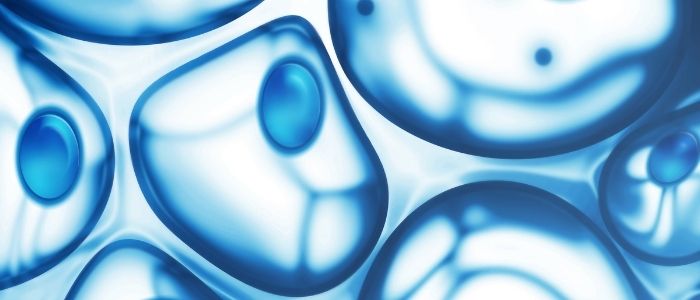
To answer the most perceptive questions, you need the most discerning tools. But when those tools don’t exist: what do you do?
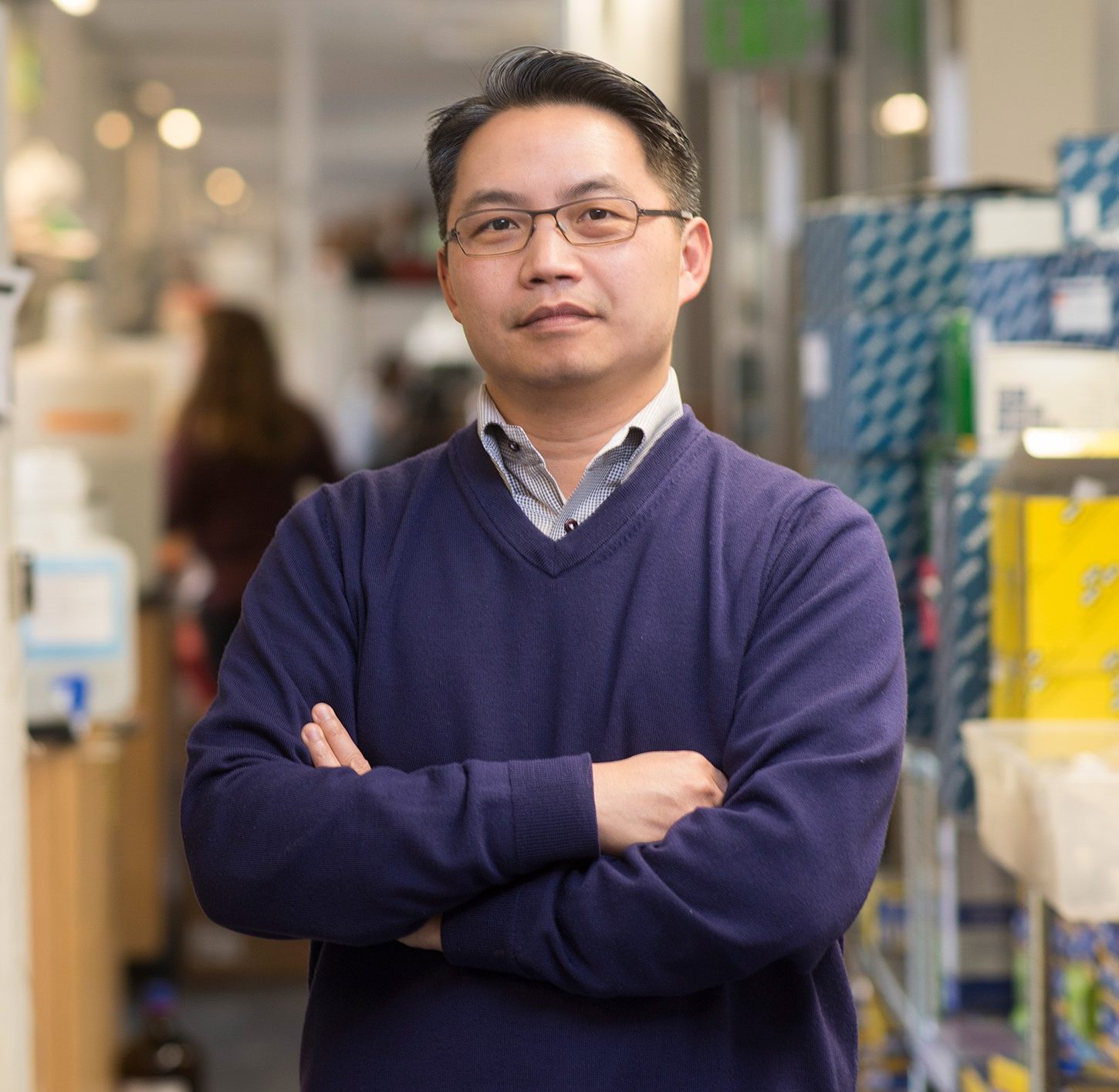
Howard Chang, Stanford University.
Due to their in-depth research into the mechanisms and control of gene expression, members of the Chang Lab at Stanford University (CA, USA) find this conundrum resting itself neatly at their door with increasing frequency. Rather than shoo it away or look for a simpler question to pursue, physician scientist and the lab’s PI, Howard Chang welcomes it inside and works with his team to create new methods with which to interrogate the human genome and better understand disease states.
Here, Chang explains the breadth of multiomic techniques he uses in this research, the diseases that his research could influence and the insight he has gained into cancer, the immunological sex biases that have been laid bare by autoimmune diseases and, more recently, COVID-19. Chang also explains how his initial dermatological training has given him a unique perspective on the future of data interpretation in multiomic studies.
You run a lab at Stanford; what does that lab focus on?
Our lab is comprised of approximately 20 scientists, ranging from postdoctoral fellows to graduate students. We’re primarily focused on understanding the hidden information contained in the human genome. Our research programs are based around biologically driven questions, in that they are attempting to understand certain disease states. However, they also have a strong thrust in technology development: a lot of questions we face have no existing techniques that can answer them.
A lot of the method development is done together with my colleague at Stanford, Will Greenleaf. We feel that these two approaches are mutually reinforcing: on many occasions, we have established a new technique and then uncovered new biology.
Are there any multiomic aspects to your studies of the genome?
That is a great question. Our research has always been driven by the principle that we want a comprehensive and unbiased description of the process or the problems that we’re dealing with, whether it’s the biological process or disease stage. For many years we have focused on genome-scale methods that can cover a biological event. And so many of these techniques turn different kinds of measurements or questions from a biochemical state to a problem that we can solve by leveraging the power and improvements in next-generation sequencing.
Most recently, our interest in gene regulation led us to open chromatin, which is a hallmark of active DNA regulatory elements, to probe the state of gene regulation. Combining studies of chromatin conformation with other modalities: that is where multiomics comes to the fore. This approach allows us to track every step in the lifecycle of gene expression.
What specific techniques are you using in these multiomic studies?
We have benefited a lot from understanding gene regulation from the perspective of open chromatin. Several years ago Greenleaf and I introduced ATAC-seq, which is a method that probes open chromatin using a transposase, which is an enzyme that copies and pastes DNA into open chromatin sites exclusively. That method led to the development of single-cell ATAC-seq, with which we can measure open chromatin states in tens of thousands of individual cells. ATAC-seq really improved the speed and scale of measurements compared to other biochemical techniques.
We’ve subsequently combined single-cell ATAC-seq with single-cell RNA-seq, a method that’s also improved in parallel. Those combinations have a lot of advantages. Currently, we are trying to integrate spatial information into our single-cell measurements, in order to simultaneously probe the epitope measurements, using CITE-seq or other related methods.
Are there any particularly interesting insights that you’ve been able to ascertain using those multiomic approaches?
I would highlight two aspects. One is that these multimodal methods allow us to move from simply observing changes towards understanding mechanisms. We often see in biological states or disease states that gene expression programs are changing, but we don’t know why. When we have ATAC-seq, we can see a prior step in the mechanism. It’s very important to link these sequential events one to another; simultaneously tracking these different modalities and measurements in the same cell allows you to do that. In some time-course measurements, we can see that the chromatin changes precede the changes in RNA expression. That, of course, is exactly what we expect, but that kind of information gives you more confidence that this is a direct mechanism and that we understand the steps involved.
Several years ago, for example, we studied the process of blood cell development. We could track different changes from hematopoietic stem cells to more restricted types of stem cells that give rise to either white blood cells or myeloid cells. And we could see the changes in chromatin and relate them to the changes in RNA.
A similar kind of phenomenon occurs when we focus on T cells – cells of our immune system that fight infections and cancer. When they’re repeatedly stimulated, T cells enter a state called exhaustion. It is an epigenetic state where they become refractory to further stimulation. Again, we can use a multi-modal approach to see the sequential changes in chromatin prior to changes in RNA and prior to changes in the protein markers that we track. Essentially, we can look under the hood and see the driving forces for each of these programs in the cell. That’s a good example in tracking changes of cell state and cell fates.
Another interesting way to use multiomics is to gain insights into cell lineage. We’ve used that approach to study the immune system. Cells in our adaptive immune system, T cells and B cells, undergo something called somatic DNA rearrangement. In this process the immune receptor genes can recombine within the cell, leading to a very large possible repertoire of alterations in the resulting genes – up to 1015 – and those changes are then inherited in all the daughter cells. This provides a trail to track the lineages of immune cell clones as they expand or contract in a disease state.
By combining RNA sequencing of the immune receptor gene loci with chromatin or ATAC-seq and other global RNA changes, we can see at the single-cell level that each of these cells is originally derived from the same lineage. In the context of cancer or cancer immunotherapy, certain clones can no longer react while others take their place and expand in prevalence. Ultimately this allows us to determine which T cells are actually fighting a cancer in the context of cancer immunotherapy.
Do you have any advice for best practices when using these techniques together?
These techniques are potentially complex and each technique has some potential pitfalls. In many cases having a reference point, a biological system where you have some context, is very helpful: just to know that the method is working as expected.
You can make this work with single-cell methods. If the system that you’re studying has reference figures derived from bulk measurements, then you can sum the results from your single-cell analyses. This sum should reproduce the bulk measurements as these have essentially come from a collection of single cells. If they do not match, then there’s some sort of disconnect or methodological issue.
A few years ago, I and many colleagues in the field put forward a set of standards, which we published in Nature Biotechnology, for epigenomic studies. These include using certain cell lines that are very well characterized to test a new method or process; making sure that they observe what people have seen before in these standard cells. These cell lines are part of the “tier one” of cell lines: they have been extensively studied, distributed to too many labs and are a part of the 1000 Genomes Project.
You’ve highlighted cancer as a disease area that your research could impact. Are there any other diseases that your work could affect?
We’re currently excited about some new findings that help explain sex differences in biology and medicine, specifically sex bias in immunity. A major thrust in my work is to generate a personal understanding of gene regulation, with the hope that this would benefit precision medicine.
We know that there are many differences between men and women in the context of immunity. Autoimmune diseases have a very strong bias towards women, four out of five patients with autoimmune diseases are female. In some diseases, like lupus, the ratio is more like nine-to-one female to male. Conversely, in the current COVID-19 pandemic, sex is the third most powerful indicator, after age and learning difficulties, of a negative outcome: men do much worse than women with COVID-19.
Our investigations of the process of X chromosome dosage compensation led us to some recent findings that the mechanism of long-term memory for gene silencing on the X chromosome is potentially more plastic than previously believed, particularly in immune cells. Essentially, this shows that females are different from males because the epigenetic silencing memory system is under continual challenge and it needs continual reinforcement to maintain the status quo.
If you could ask me for one thing to help with your next breakthrough or insight into gene regulation, what would it be?
Increasingly, we need new advances in computation and ways of visualizing and interpreting these data. As we start to make these multiomic measurements in scores of single cells, we have a huge amount of data and it’s becoming difficult to view it all in a way that you can process. Imagine skimming through a 10,000-page dossier and trying to process all the information at once: you can’t keep it all in your head and make sense of it.
But it’s a higher-level issue than simply displaying all the information or compressing it. Instead, it’s asking how you can extract meaning and insight from that data. I would love some help with that and am actually looking for colleagues to contribute to this our area.
To draw an example from my own training as a dermatologist; when you learn about skin diseases you start to learn what features to look for, some of which have more meaning than others. This is similar to a trained pathologist looking for certain patterns in slides of cells under a microscope. Often, a very low resolution is more informative than focusing on individual cells. It’s the overall pattern or features that you’re looking for. We need to be able to these data in a way that is akin to spotting these features on someone’s skin; to extract information on a macro scale without delving into the individual data points.