Machine learning algorithm predicts cell growth
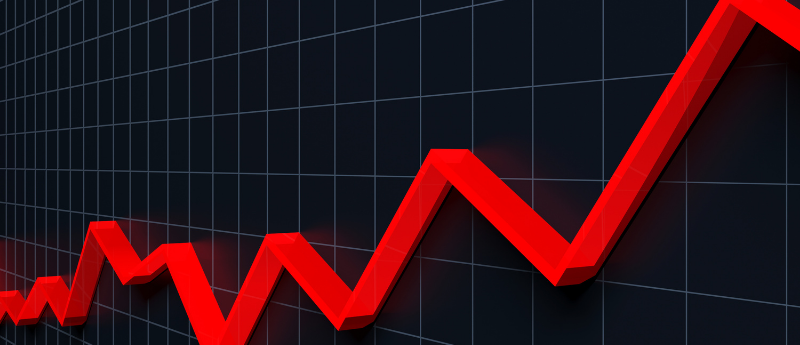
Cell growth seems like a simple concept, but scientists still have a lot to discover about this mechanism. Now, researchers have developed a machine learning algorithm to predict the size that an individual cell will grow to.
By using relatively new technology in the form of a deep neural network, the team, from The University of Tokyo Institute of Industrial Science (Japan), could enable a computer to accurately forecast the size of a cell and how it grows and divides. The work has important implications in quantitative biology and could be used in industry for producing medicines.
Mathematical models have been used to attempt to answer many biological questions, with varying degrees of success. The reason for these varying degrees is that biology isn’t too inclined to conform to rules set in these mathematical models for predicting the future, and is full of hidden complexities that make up the living world – the variety of life.
Many models make assumptions about a situation to give a suitable answer, but these assumptions do not necessarily match up to the real-life biological processes and the quirks that come with them.
By using a neural network and machine learning algorithm the computational model can learn over the course of its analyses, it has the flexibility to recognize different patterns and can adapt the model to reflect these real-life nuances. In this study, the algorithm could measure the size of a single cell over a period of time, then use this data to predict the size of the cell in the future.
Deep learning for enhanced drug design
Every drug that makes it to the clinic has undergone extensive testing to ensure its efficacy and safety. However, often the safety of a drug depends not on the drug itself but on the metabolites that it produces when it is broken down.
“In biology, simple models are often used based on their capacity to reproduce the measured data,” stated first author on the paper, Atsushi Kamimura (The University of Tokyo). “However, the models may fail to capture what is really going on because of human preconceptions.”
To test their machine learning algorithm the researchers collected data from Escherichia coli bacteria and Schizosaccharomyces pombe yeast cells, each suspended in a microfluidic channel at different temperatures. The results were plotted as the size of the cells was measured over time, producing a graph in a ‘sawtooth’ pattern. The size increases leading to peaks at which the cell then divides, producing troughs.
Traditionally, biologists have used two models to predict cell size and cell division; the ‘sizer’ model, which uses the absolute size of the cell and the ‘adder’ model, which calculates the division points based on the increase in cell size since birth. In this study, the machine learning algorithm was found to support the ‘adder’ model more closely but, using the additional understanding of biochemical reactions and signaling cascades that are involved in cell growth.
“Our deep-learning neural network can effectively separate the history-dependent deterministic factors from the noise in given data,” senior author Tetsuya Kobayashi (The University of Tokyo) concluded.
The accuracy of the machine learning algorithm to determine these biological processes is remarkable and the authors hope the technology can be used in the future for more precise control of microorganisms used to produce drugs.