Democratizing microscopy and diagnostics and developing digital holography: an interview with Aydogan Ozcan
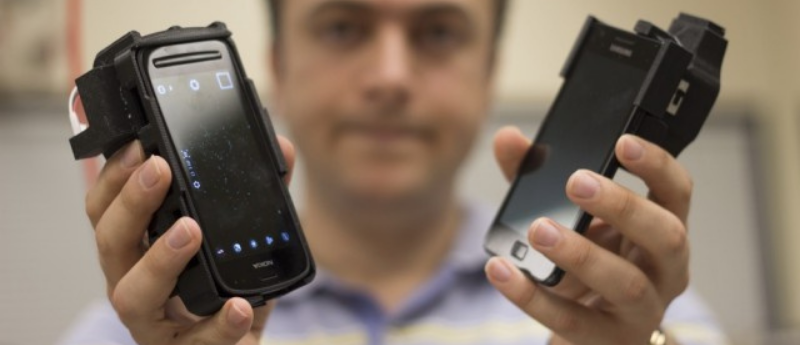
Aydogan Ozcan (left) is the Chancellor’s Professor and Volgenau Chair for Engineering Innovation at the University of California, Los Angeles (CA, USA) where he leads the Bio- and Nano-Photonics Laboratory. He is also a Professor at the Howard Hughes Medical Institute and the Associate Director of the California NanoSystems Institute.
Here, he discusses his work in the democratization of microscopy and detection technologies and reveals the latest advances in digital holography and explains how advances in AI have impacted this technique.
What is the focus of your work with the Ozcan Lab?
My lab is composed of 3 sub-areas:
- Sensing: point-of-care sensors, mobile-phone enabled sensors, field-based sensing and measurement systems with applications in mobile health and telemedicine, environmental monitoring (for example, air/water quality sensing)
- Computational microscopy, which includes deep learning-enabled microscopy, holography, lensless imaging, on-chip microscopy, 3D microscopy, imaging flow-cytometry, among others.
- Optical computing and inverse design: diffractive networks, diffractive optical processors, and deep learning-designed free-space optics
While conducting exciting, cutting-edge applied research on photonics and optics, we also train the next generation of engineers, scientists and entrepreneurs through our research programs. Some of our trainees have started up their own labs in the United States, Europe, China and other parts of the world or gone on to lead labs in industry.
What is the aim of your sensing and point of care technology focus?
My research on computational imaging, mobile sensing and diagnostics has created widely-scalable mobile technologies for blood analysis, sensing pathogens and toxins in bodily fluids, food and water samples, diagnosis of infectious diseases, screening of antimicrobial resistance, pathology analysis, as well as particulate matter and bio-aerosol detection for air quality measurements. Altogether, these technologies have the potential to dramatically increase the reach of advanced biomedical technologies to developing countries and resource-limited settings. My work broadly helps to democratize biomedical measurement science by enabling advanced measurements to be cost-effectively performed even in field settings, using mobile instruments powered by computational optics and machine learning.
My lab was one of the first teams that utilized the cellphone as a platform for advanced measurements, microscopy and sensing, covering various applications. For example, we were the first group to image and count individual viruses and individual DNA molecules using mobile phone-based microscopes. We were one of the first groups to utilize the smartphone as a platform for quantitative sensing, for example, quantification of lateral flow tests. Our mobile diagnostic test readers are still being used in the industry through a licensee of some of our patents. Lucendi (CA, USA), a start-up that I co-founded, has commercialized a mobile imaging flow cytometer for water quality analysis, including screens for toxic algae blooms.
As another example, our team has introduced the first point of care sensor designed by machine learning that runs and makes decisions based on a neural network. This vertical flow assay can look at >80 immunoreactions in parallel. We have shown its efficacy for detecting early-stage Lyme disease patients based on IgG and IgM panels (profiling the patient’s immunity), published in ACS Nano. We are also considering a similar approach for COVID-19 – especially important to understand e.g., the efficacy of vaccines and when a booster shot is needed.
Please could you explain what digital holography is?
Digital holography is a method that uses the interference of light to reconstruct the phase and amplitude information encoded in optical waves or specimen. This permits label-free imaging of cells or tissue samples, for example, revealing various structural details that would normally be invisible to or hard to see by a brightfield microscope, unless they are labeled by external tags. My team has made various seminal contributions to digital holography and its applications for microscopy, sensing and telemedicine over the last 15+ years.
How has AI influenced the development of digital holography in the last 5 years?
In 2017, my lab demonstrated the first use of deep neural networks for holographic image reconstruction and phase recovery. We demonstrated that a convolutional neural network (CNN) can learn to perform phase recovery and holographic image reconstruction after appropriate training. This deep learning-based approach provides a fundamentally new framework to conduct holographic imaging by rapidly eliminating twin-image and self-interference-related spatial artifacts.
These artifacts have been a challenging reality of holography since its invention by Dennis Gabor, which led to the Nobel Prize in Physics in 1971. Compared to existing holographic phase-recovery approaches, this neural-network framework is significantly faster to compute and reconstruct sincerely improved phase and amplitude images of the objects using a single hologram. Therefore, it requires fewer measurements in addition to being computationally faster. Remarkably, this deep-learning-based twin-image elimination and phase recovery have been achieved without any modeling of light-matter interaction or a solution of the wave equation.
After this 2017 LSA publication, our team has further expanded these results in many unique ways. In a paper published in 2018 by the Optical Society, we demonstrated an innovative application of deep learning to significantly extend the imaging depth of a hologram. We demonstrated a CNN-based approach that simultaneously performs auto-focusing and phase-recovery to significantly extend the depth-of-field (DOF) in holographic image reconstruction.
To do this, a CNN is trained by using pairs of randomly de-focused back-propagated holograms and their corresponding in-focus phase-recovered images. After this training phase, the CNN takes a single back-propagated hologram of a 3D sample as input to rapidly achieve phase-recovery and reconstruct an in-focus image of the sample over a significantly extended DOF. Furthermore, this deep-learning-based auto-focusing and phase-recovery method is non-iterative, and significantly improves the algorithm time-complexity of holographic image reconstruction.
How has the introduction of deep neural networks improved the accuracy and depth of insight obtained by digital holography?
Another breakthrough, in my opinion, at the intersection of deep learning and holography followed in a preprint article from my team, posted in 2018. In this article, we introduced a deep neural network to perform cross-modality image transformation from a digitally back-propagated hologram corresponding to a given depth within the sample volume, into an image equivalent to a brightfield microscope image acquired at the same depth. Because a single hologram is used to digitally propagate two different sections of the sample to virtually generate brightfield equivalent images of each section, this approach bridges the volumetric imaging capability of digital holography with speckle- and artifact-free image contrast of brightfield microscopy. After its training, the deep neural network learns the statistical image transformation between a holographic imaging system and an incoherent brightfield microscope, and intuitively it brings together ‘the best of both worlds’ by fusing the advantages of holographic and incoherent brightfield imaging modalities.
For this holographic to brightfield image transformation, we used a generative adversarial network (GAN), which was trained using pollen samples imaged by an in-line holographic microscope along with a brightfield incoherent microscope (used as the ground truth). After the training phase, which only needs to be performed once, the generator network blindly takes as input a new hologram (never seen by the network before) to infer its brightfield equivalent image at any arbitrary depth within the sample volume. We experimentally demonstrated the success of this powerful cross-modality image transformation between holography and brightfield microscopy. We also demonstrated that the deep network also correctly colorizes the output image, using an input hologram acquired with a monochrome sensor and narrow-band illumination, matching the color distribution of the brightfield image.
This deep learning-enabled image transformation between holography and brightfield microscopy replaces the need to mechanically scan a volumetric sample, as it benefits from the digital wave-propagation framework of holography to virtually scan through the sample. Each one of these digitally propagated fields is transformed into brightfield microscopy equivalent images, exhibiting the spatial and color contrast expected from an incoherent microscope, as well as the depth-of-field.
How have you adapted to the increasingly virtual nature of lab research?
Approximately 3 years ago, my lab published a paper that introduced a deep learning-based method to ‘virtually stain’ autofluorescence images of unlabeled histological tissue sections, eliminating the need for chemical staining. This technology was developed to leverage the speed and computational power of deep learning to improve upon century-old histochemical staining techniques which can be slow, laborious and expensive. In this paper, we showed that this virtual staining technology, using deep neural networks, is capable of generating highly accurate stains across a wide variety of tissue and stain types. It has the potential to revolutionize the field of histopathology by reducing the cost of tissue staining, while making it much faster, less destructive to the tissue, more consistent and repeatable.
Since the publication of our paper, we have had a number of exciting developments moving the technology forward. We have continued to find new applications for this unique technology, using the computational nature of the technique to generate stains that would be impossible to create using traditional histochemical staining. For example, we have developed a ‘digital staining matrix’ that allows us to generate and digitally blend multiple stains using a single deep neural network, by specifying which stain should be performed on the pixel level.
Not only can this unique framework be used to perform multiple different stains on a single tissue section, it can also be used to create micro-structured stains, digitally staining different areas of label-free tissue with different stains. Furthermore, this digital staining matrix enables these stains to be blended together, by setting the encoding matrix to be a mixture of the possible stains. This technology can be used to ensure that pathologists can receive the most relevant information possible from the various virtual stains being performed.
This work was published in 2020 and opened up the path for a very exciting new opportunity: stain-to-stain transformations. This enables the transformation of existing images of tissue biopsy stained with one type of stain into many other types of stains, almost instantaneously. This stain-to-stain transformation process takes less than one minute per tissue sample, as opposed to several hours or even more than a day when performed by human experts. And, this speed differential enables faster preliminary diagnoses that require special stains, while also providing significant savings in costs.
What is your main focus as you look to the future?
Motivated by the transformative potential of our virtual staining technology, we have also begun the process of its commercialization and founded Pictor Labs (CA, USA), a new Los Angeles-based start-up. Pictor in Latin means ‘painter’ and at Pictor Labs we virtually ‘paint’ the microstructure of tissue samples using deep learning. In the second half of 2020, we successfully raised seed funding from venture capital firms including M Ventures (a subsidy of Merck KGaA), Motus Ventures, and private investors.
Through Pictor labs, we aim to revolutionize the histopathology staining workflow using this virtual staining technology, and by building a cloud computing-based platform that facilitates histopathology through artificial intelligence, we will enable tissue diagnoses and help clinicians manage patient care. I am very excited to have this unique opportunity to bring our cutting-edge academic research into the commercialization phase and look forward to more directly impacting human health over the coming years using this transformative virtual staining technology.