Ask the Experts: DNA methylation studies
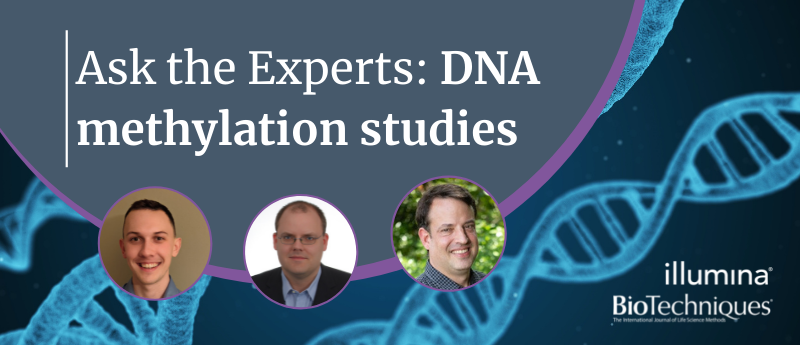
DNA methylation is an epigenetic modification characterized by the addition of a methyl group to a DNA molecule. As DNA methylation is implicated in both normal biological processes, such as development and aging, and disease states like cancer and neurological disorders, DNA methylation analyses provide valuable insights into health and disease.
In this ‘Ask the Experts’ feature, Per Hoffman (Life & Brain Genomics; Bonn, Germany), Robert Hillary (University of Edinburgh; UK), and Jörg Tost (Centre National de Recherche en Génomique Humaine; Evry, France), discuss their perspectives and top tips for DNA methylation studies.
Download this feature as a PDF
DNA methylation questions:
- There are different types of epigenetic markers. When would you consider DNA methylation?
- What can you measure with DNA methylation?
- It is sometimes difficult to tell correlation from causation in DNA methylation studies. Which measures can you take to cope with that?
- What do you need to think of with regards to samples for DNA methylation studies?
- What are your recommendations regarding replicates (technical and biological) and controls?
- Do you do intra-specimen comparisons (healthy state vs disease state, longitudinal etc.) and how do you establish a baseline for normal/healthy?
- The raw data consists of intensity files from fluorescent labeling. What are the next steps in data processing?
- What advice can you give to someone who considers embarking on a DNA methylation study for the first time?
There are different types of epigenetic markers. When would you consider DNA methylation?
Per Hoffman (PH): Methylation is the most stable form of epigenetic modification and is therefore particularly suitable in terms of detecting the long-term effects of environmental influences. The key focus of our research is the role of epigenetics in multifactorial diseases. In these disorders, both external influences and genetic factors play an important role. Examples of such external influences include adverse environmental influences during childhood. In the case of psychiatric disorders, such influences continue to exert their effects on mental health beyond childhood and into adulthood. DNA methylation studies are particularly suitable for detecting long-term effects of this nature at the molecular level. We also operate as a core facility/service provider. In this context, we use the characterization of methylation status to identify causative pathways, as well as in the development and application of cancer classifiers. Among others, we apply this approach to elucidate epigenetic clocks in neurodegenerative disease, and in the investigation of tumors. DNA methylation thus has a plethora of potential applications in the study of epigenetic phenomena.
Robert Hillary (RH): DNA methylation levels can be measured across hundreds of thousands of CpG sites using microarrays, including Illumina’s Infinium™ HumanMethylation BeadChips. These technologies are cost effective and high throughput, which permits their use in large-scale studies and population biobanks. There are also high-throughput methods for measuring other epigenetic markers such as histone modifications, chromatin marks and hydroxymethylation, and these technologies continue to be refined. However, DNA methylation microarrays are, at present, the most amenable to large-scale studies.
DNA methylation is dynamic and influenced by genetic and environmental factors. It provides a molecular mechanism through which gene expression can be regulated in cell- and tissue-specific manners. By contrast, genetic risk factors are fixed and only estimate lifetime disease liability. Therefore, DNA methylation data are important for identifying (i) mechanisms underlying complex traits and disease and (ii) biomarkers for these traits. DNA methylation also plays an important role in X-chromosome inactivation, genomic imprinting and silencing of transposable elements. DNA methylation data are important for understanding the mechanisms underlying these cellular and molecular events.
Jörg Tost (JT): DNA methylation has – as a DNA-based modification – a number of technical advantages, such as stability in biological samples. It is relatively easy to analyze using one of the many methods available for the analysis of genetic variation once the DNA methylation state has been translated into a sequence-based variation, most commonly by sodium bisulfite treatment. Furthermore, the possibility to start from relatively small amounts of DNA, as the converted DNA can be easily amplified after bisulfite conversion using PCR (not before as the DNA methylation marks are lost during conventional PCR), and the limited dynamic range – between 0 and 100 % of alleles in the sample – make DNA methylation a molecular level of interest for many applications. Several benchmarks have also demonstrated the maturity of both locus-specific and genome-wide technologies, and many technologies for DNA methylation analysis are scalable and more amenable to the analysis of very large cohorts compared to histone modifications and chromatin accessibility, for example. Additionally, DNA methylation-based biomarkers have been approved by regulatory agencies, which is an important step for their implementation into clinics. DNA methylation is therefore well-suited for analysis in both research settings and clinical applications.
Compared to other epigenetic modifications, DNA methylation shows a reduced plasticity, meaning that the changes are slower to occur but also more stable and the measurement of DNA methylation is associated with less random noise. DNA methylation can be considered as the epigenetic modification keeping the cellular epigenome in its altered state, providing a more robust biomarker with many applications including the analysis of methylation of cell-free circulating DNA in various body fluids.
What can you measure with DNA methylation?
PH: First, DNA methylation can be used to measure epigenetic age. Since interest in healthy food and healthy living is increasing in the hope that this may render people biologically younger than their chronological age, epigenetic clocks are now a major focus in both research and direct to consumer testing.
Second, DNA methylation can be used to identify epigenetic classifiers. In terms of glioblastomas, for example, the availability of epigenetic classifiers over the past 3 years has led to a major change in neuropathological classification. Previously, diagnosis relied on histopathology. Now, however, the use of methylation classifiers is standard practice, and this improved diagnostic tool has resulted in more than 30% of diagnoses being changed. Researchers are in the process of developing classifiers for other diseases, for example leukemia. The leukemia classifier has the potential to improve diagnostics at the same level as the glioma classifier.
I believe there will be much more to come. We have only been doing epigenetic microarrays in high numbers for less than 5 years now, and since science needs time and samples sizes, I believe we will see more showing up over the coming years.
RH: Epigenome-wide association studies (EWAS) and differentially methylated region analyses are used to uncover genomic loci associated with phenotypes. It is important to note that association studies require correction for the multiple tests that are performed. Machine learning approaches are also applied to DNAm data to develop epigenetic predictors of human traits, such as biological age measures.
Candidate gene studies examine the relationship between DNA methylation across a specific gene and an outcome of interest. However, large subsets of CpG sites co-vary with one another. The high degree of inter-correlation structure can, in theory, inflate test statistics from gene-wise studies. Candidate gene methylation studies are possibly better suited to follow-up studies as opposed to their use as discovery tools.
JT: There are so many different applications in which DNA methylation analysis might be useful that my list is clearly incomplete.
Especially in the cancer field it has been shown that DNA methylation changes are present in pre-neoplastic lesions and early tumors, making them a prime target for the early diagnosis of the disease. As we can also analyze DNA methylation in cell-free circulating DNA (liquid biopsy), this can be used to detect abnormal DNA methylation and due to the cell-type specificity determine its origin, as proposed for example by Grail Inc. The same characteristics also enable the localization of the primary tumors when only metastatic lesions have been detected and adapt the treatment to the primary tumor rather than the metastasis. DNA methylation profiles also improve classification of the disease and prediction and/or monitoring of the response to cancer treatments, well illustrated by the importance of the DNA methylation status of the MGMT promoter for the response to temozolomide in glioblastoma. While many of the applications have been focusing on cancer, I strongly believe that there is an equally important if not even greater potential for DNA methylation in many other complex diseases, especially in those where do we not have strong genetic drivers. Furthermore, DNA methylation can be regarded as the memory of our exposure including both external lifestyle factors (such as food, smoking and other pollutants), but also internal factors such as hormonal or stress exposure. DNA methylation analysis might help us to identify how these factors will change cellular homeostasis and eventually might lead to the occurrence of disease.
It is sometimes difficult to tell correlation from causation in DNA methylation studies. Which measures can you take to cope with that?
PH: This is dependent on the study in question. On the one hand, researchers must control as accurately as possible for factors with a known influence on methylation. Such factors include age, gender, smoking and the use of medications. To control for the influence of these factors, they must have been accurately documented during the course of the study. For epigenetic studies, this should therefore be taken into account – if possible – during the study design process. Causal effects can also be distinguished from correlations through the inclusion of genetic data and the application of special statistical procedures. Longitudinal studies, in which methylation changes can be related to changing environmental factors in a prospective cohort, also offer advantages.
RH: DNA methylation data are susceptible to confounding influences and reverse causation. Analogous to the randomized control trial design, Mendelian Randomization (MR) is a commonly used approach to assess causality in epidemiological studies. In MR, one or more genetic variants (termed instruments) that robustly associate with an exposure variable (i.e. risk factor) are used to proxy for the exposure. Associations between genetically-predicted levels of the exposure and outcome variables are then tested. Confounding variables should not influence the germline genetic variants that are used as instruments. Further, genetic variants are non-modifiable and are therefore not susceptible to reverse causation. Genetic factors that correlate with CpG methylation are known as methylation quantitative trait loci (methylation QTLs or mQTLs). Databases of mQTLs are publicly available, the largest of which comes from the recent GoDMC consortium. An excellent resource on MR was also recently developed by the MRC Integrative Epidemiology Unit at the University of Bristol (UK).
Longitudinal data can help to assess causality. Bayesian analyses of genetic colocalization (such as the popular R package coloc) can also support causal inference. Data from complementary methods can be triangulated with evidence from the literature to support a likely model of causality, which should be replicated in external datasets.
JT: This is of course a very important point, which is not easy to address, and we have to admit that most associations so far reported between phenotypes and DNA methylation are purely correlative. There are statistical approaches such as MR that can be used to provide evidence, if the effects of DNA methylation at CpGs associated with a given trait on a measurable phenotype are mediated through DNA methylation. Additional information can be obtained through longitudinal sampling in large population-based cohorts. Of course, biological material needs to be sampled in cells of relevance to the questions, as DNA methylation is cell-type specific as discussed below in more detail. Such cohorts could provide some clues if DNA methylation precede and might be driving the onset of a phenotype (e.g. a specific disease) and has a probability to be causally implicated in the process, although it does not guarantee this. A good example of this is smoking-related DNA methylation changes, which have been shown to be functionally implicated and at least contributing to the development of lung cancer in several studies.
Recent technological developments such as epigenetic editing, in which a guide RNA and the d(ead)Cas9 complex fused to the catalytic core domain of an epigenetic enzyme such as a DNA methyltransferase and or demethylase, can be used to specifically methylate or demethylate a specific genomic region and thereby functionally validate the impact of DNA methylation on a phenotype under investigation.
What do you need to think of with regards to samples for DNA methylation studies?
PH: Obviously, methylation can easily be tested using DNA obtained from blood or saliva samples. However, tissue specificity is an important issue in methylation studies. For example, blood-brain comparisons only have a correlation of around 30%. Therefore, while the use of blood as a biomarker for brain phenotypes may work well for very robust methylation changes, it will be ineffective in the case of subtle changes, or in the establishment of classifiers, for example for cancer classification.
Adequate DNA quantity is essential, because low amounts of DNA can, like in next-generation sequencing (NGS), lead to less complex libraries. As a result, only very robust epigenetic changes are seen, while low abundance epigenetic changes are lost. In general, a methylation microarray detects methylation changes of 20–30% and above. If greater sensitivity is required, NGS methods must be used, with all their attendant challenges.
RH: FFPE compatibility, consistency across samples, inhibitors, bisulfite treatment
The multiplexed detection of CpG methylation rests on genotyping bisulfite-converted genomic DNA. First, you must consider if your tissue is compatible with bisulfite conversion and sequencing. Normal and formalin-fixed paraffin-embedded (FFPE) samples are compatible with Infinium workflows. However, a modified version of the workflow is available for FFPE samples, which utilizes a FFPE DNA Restoration solution to restore degraded FFPE DNA to an amplifiable state. Whole genomic DNA samples should be normalized to a specified amount to ensure consistency. Cellular heterogeneity in your tissue of choice is also of paramount importance in DNA methylation studies. Methylation-based models for estimating cellular compositions have been developed for cord blood, brain and buccal samples. Reference-free methods are also available to adjust for cellular composition and assume that the error structure is associated with cellular heterogeneity.
Demographics and lifestyle
Demographic factors including age, sex and ethnicity associate with variation in DNA methylation patterns. In a given study, stratification by demographics such as age group and sex is desirable. However, numerous stratifications are not always possible in small or modest sample sizes. Ensuring that the sample is homogenous with respect to demographic variables circumvents the issue but precludes generalizability of the results to other populations. Furthermore, the majority of DNA methylation studies include individuals of European ancestry. There is a stark need for greater diversity in epigenetic epidemiology and health outcomes research as a whole.
Lifestyle factors should be measured to permit statistical adjustment in regression models. Furthermore, estimates of lifestyle factor exposure such as smoking can suffer from systematic error including recall bias (i.e. an inaccurate report of previous exposure) and non-random missing data. Whereas some sources of bias cannot be eliminated, it is important to report and appraise biases when interpreting your findings.
JT: I think that the selection of samples is crucial for the success of a study. It should be kept in mind that DNA methylation patterns are cell-type specific, and the relevance of the biological material sampled to the question needs to be considered. Therefore, I have to ask myself if the biological material which I have at my disposition is suited to address the question of interest. For example, does it make sense to analyze Alzheimer’s disease in circulating blood cells? Maybe if you are trying to detect the low-level inflammation associated with the disease, but to see changes related to the initiation of neurodegeneration, probably brain samples and perhaps even isolated neurons might be required. Will the sample allow me to detect true DNA methylation changes or is it likely that I will only see changes in the proportions of different cell types contained within the sample and that actually the proportion of cells varies between groups? For example, I will detect DNA methylation differences between people with and without a viral infection in whole blood or peripheral blood mononuclear cells, but these mostly reflect an increase in inflammatory cells in the bloodstream. Controlling for cell composition will remove most of the observed DNA methylation changes. Therefore, in most of our studies we analyze DNA methylation in magnetic activated, or fluorescence activated, sorted cell populations, which facilitate the detection of DNA methylation changes in well-defined cell populations. Some compromise might be needed though as the required amount of input material for the analysis technology needs to be kept in mind.
What are your recommendations regarding replicates (technical and biological) and controls?
PH: First, if a methylation array is to be used, this analysis should be performed by a large service provider, as this is Illumina’s most complicated array product. It is a mixture between a normal genotyping array and quantitative analysis, and you may want people to perform the analysis who are trained and are regularly using this array. If a group plans to establish the analysis itself, but only receives samples every 2 weeks, they will not achieve the required experience and quality. Secondly, involving a large service provider will probably preclude the need for technical replicates, as they use standardized and automated procedures. The sample-dependent and -independent controls on the microarray can also be used to correct for batch effects. In my opinion, the routine use of technical replicates is unnecessary.
RH: A primary source of technical variation in DNA methylation studies involves batch effects. Batch effects occur when differences between groups of samples are introduced during handling and/or measurement. Systematic measurement errors can occur between samples on a given chip (8 samples per chip), the chips or the plates (12 chips per plates). Different times of measurement, sites in multicentre studies and experimenters can also introduce batch effects.
Technical replicates involve repeated analysis of the same biological sample. Genome-wide methylation patterns should correlate strongly across technical replicates. Further, probes that show poor concordance across technical replicates may be removed. Biological replicates are different samples measured across multiple conditions, e.g., six different samples across six arrays. Replicates can help to detect outliers. Where possible, samples from the same subject (i.e. longitudinal measures), matched sets (matched case and control) or related groups should be analyzed within the same batch in order to prevent unwanted bias due to between-assay variability. Comparison groups should be randomized across batches. Experimenters should be also blinded to sample types.
JT: The Infinium methylation technology is very mature and robust. If large DNA methylation differences are expected to be observed, such as in the analysis of cancer samples compared to healthy controls, and if samples to be analyzed are of good quality and available in sufficient quantity as recommended in the protocol, the study will profit more from including biological replicates rather than technical replicates. For samples which are of lesser quality such as FFPE conserved samples, it might be worth performing two separate restoration steps and analyze the samples in parallel as some technical differences might occur. However, for the detection of small DNA methylation differences, which might be observed in complex disease and especially in exposure studies, a careful design including multiple technical replicates is helpful. This will assist in the assessment of the presence, but also in the efficiency of the correction between batch effects that might be observed between multiple runs, samples plates and/or position on the BeadChip. To avoid confounding influences in the later statistical analyses, it is also important to randomize all potential confounding factors and factors which shall be adjusted for over the entire study and the employed BeadChips using computational tools such as the R package OSAT.
On the other hand, we have previously included several control samples, such as completely methylated and unmethylated DNA, as well as a cell line DNA incorporated in each run. This allowed us to continuously monitor the quality of the results, changes in fluorophore intensities, the percentage of successfully analyzed CpG positions etc.
While still useful for projects with samples of unknown or suboptimal quality, or applications of the Infinium array other than the recommended use such as DNA methylation analyses of non-human primates, this approach was not very cost effective as we hardly ever encountered a problem with samples analyzed by the Infinium arrays and therefore we dropped the controls in favor of additional technical and biological replicates. The control probes included on the array are more than sufficient to assess the quality.
Do you do intra-specimen comparisons (healthy state vs disease state, longitudinal etc.) and how do you establish a baseline for normal/healthy?
PH: That is an interesting question, since again it involves multiple issues. Yes, we do, of course, perform comparisons of healthy versus disease states. We also conduct longitudinal studies, as it is interesting to determine whether changes in methylation occur over time. For example, this approach can be used to determine differences on the epigenetic level between the normal ageing process and the ageing process in individuals with neurodegenerative disorders.
When performing EWAS, the epigenetic baseline is defined as the average methylation value at CpG sites in the control group. If it is a longitudinal study, it is the timepoint of the first epigenetic analysis in the control group. Larger sample sizes facilitate the establishment of a good baseline, since the effects of the environment and the genetic background are averaged.
RH: Intra-sample correlations are useful for examining longitudinal (i.e. age-related) trajectories in CpG methylation. It is also possible to identify CpG sites that show significant changes pre- and post-treatment with an intervention of interest, or between healthy and diseased tissue. Ideally, these samples would be processed contemporaneously and randomized across batches (e.g. samples pre- and post-treatment). However, this is often not feasible for longitudinal and follow-up studies. We can estimate general trends with respect to changes in CpG methylation over time or post-intervention. However, individual trajectories may differ from the general trend.
JT: Yes, we do this quite frequently, for example if you would like to assess the effect of a treatment on a patient or a specific cell-type, it is much more powerful to use the pre-treatment sample of the same patient, than a group of unrelated controls. As discussed, DNA methylation is influenced by so many factors, including age, that we all have a different baseline and treatment induced changes might only slightly alter the DNA methylation changes, making it hard to detect in the presence of substantial variation within each group. Monitoring DNA methylation during clinical trials using samples that have been collected for epigenetic analyses might provide new leads on patient stratification and mechanisms of resistance. DNA methylation analysis has a yet largely unexploited potential for companion diagnostic tests. One of the greatest potentials lies within the early detection of disease, both in cancer, but also autoimmune, inflammatory, metabolic or neurological diseases. Population-based, longitudinally collected samples with sufficient follow-up time will be very powerful to detect and replicate early epigenetic changes and address their specificity and sensitivity in the presence of multiple other phenotypes.
The raw data consists of intensity files from fluorescent labeling. What are the next steps in data processing?
PH: The first step is technical quality control. Here, the sample-dependent and -independent controls on the microarray are examined to determine whether the bisulfite conversion was successful, that the staining was consistent with the specifications and to confirm that no sample mix ups have occurred. Here, documented gender is always compared with the gender that is indicated by the array.
Tools such as minfi are then used to analyze the methylation signals. Color corrections between the Infinium I and II probes are performed. In the case of blood samples, a decomposition of blood cell types will be performed. Again, adjustment must be made for the bisulfite conversion rate. In addition, the p95 thresholds are examined to determine whether a sufficient number of probes have an above-background signal. This work involves multiple steps, which renders the process quite complicated. Once these technical steps have been completed, the samples are forwarded to our bioinformatic partners for a statistical evaluation of the readouts.
RH: Samples and probes that show poor performance must be removed and this can be based on a number of criteria. For instance, probes are often removed if they were reliably detected in less than a critical threshold (such as 95%) of samples. Normalization methods are available to minimize the influence of technical variation. Between-array normalization minimizes technical variability between samples on different arrays (e.g. dasen, pQuantile). Within-array normalization corrects for dye and probe type biases (e.g. SWAN, noob, BMIQ). The influence of batch effects can be minimized by using approaches such as ComBat, Remove Unwanted Variation (RUV) and Surrogate Variable Analysis (SVA).
At the time of writing, the most recent Infinium array is the HumanMethylationEPIC BeadChip (‘EPIC’) array, released in 2016. This array measures ~850,000 CpG sites, which is an increase from the previous array (HumanMethylation450K BeadChip, also known as ‘450K’). Whereas some studies employ both arrays, others will use just one. This creates the need for pipelines to appropriately harmonize data derived from the arrays. Recently, Vanderlinden et al. (2021) proposed a harmonization workflow that covers normalization, probe quality control and filtering, batch effect adjustment and genomic inflation control.
JT: There are a number of easy-to-use and freely available bioinformatic pipelines for the analysis of methylation BeadChips, which are actively maintained by groups of motivated researchers including ChAMP, RnBeads, SeSAMe and ENmix, just to name a few which have been widely adopted by the users. An article published in Methods offers an overview of some of the most widely used pipelines. These tools will allow you to perform the removal of probes known to be problematic, for example if they are influenced by genetic variation such as SNPs or targeting multiple locations in the human genome, correct for the bias due to the use of fluorescent dyes, as well as perform a background correction. Most pipelines also contain a number of functions allowing you to perform a quality control of the data using partly the multiple quality control probes incorporated into the array and detect bad quality samples or samples that behave very differently (outliers). They can also detect technical biases such as batch effects and propose to correct for them, as well as different methods to correct for the shift between Infinium I and Infinium II probes, which are based on a different analytical approach and display a different dynamic behavior. After that your data is ready for most differential DNA methylation analysis using either single CpGs or differentially methylated regions, which can then be further annotated and interpreted for their biological meaning.
What advice can you give to someone who considers embarking on a DNA methylation study for the first time?
PH: First, choose a specialized service provider/core facility. Second, consult experienced researchers and bioinformaticians in order to ensure that your study design is appropriate for a methylation analysis. Epigenetic studies are costly and complicated, and these two steps are essential to avoid investing time and money in the generation of suboptimal data.
RH: Core laboratories and service providers can be invaluable in setting up DNA methylation studies. They may be able to run the analyses or provide advice if you are planning to carry out the analyses, e.g. advice on sample storage, processing, randomization schemes and technical aspects of the workflow. Bioinformatics and statistics core services can support data processing and quality control, as well as provide advice on the appropriateness of downstream statistical analysis plans. Be sure to check which approved repositories best suit your summary statistics so that you can publish these data in an open access and transparent manner. There are also several excellent reviews on DNA methylation study design. The list I will supply is non-exhaustive and I sincerely apologize if I have missed out on an excellent review. I recommend reviews from Michels & Binder (2018), Singer (2019) and Mansell et al. (2019).
JT: Do not be afraid of launching yourself into DNA methylation analysis, which might give you a new perspective on the biological or medical question you are investigating. The technology works very well if you have samples of correct quality and most genotyping platforms will be able to perform the experiments for you. There are many user-friendly pipelines for data analysis available for which only some basic knowledge of R is required for the analysis, and there a large number of tools to perform quality control, assess the data structure and analyze your data. There are many datasets with both raw and analyzed data available, which allow you to train yourself on these data-processing tools. It is nonetheless important to reflect well on the study: 1) do my samples allow me to answer the question I am interested in? 2) how do I correct for heterogeneous cellular composition? It is also important to evaluate the statistical power based on sample size and expected DNA methylation differences and to make sure the experiments are performed in a way that there is no bias between batches (very important: do not run all cases in one batch and all the controls in the second!). If you work with a platform, ensure you discuss your project and the required technical replicates to allow an efficient correction of any bias later on.
These are very exciting times and I think that there is enormous potential for epigenetic modifications in general and DNA methylation in particular to contribute to the individualized management of patients and personalized medicine. However, there are many things we still need a deeper understanding of to make optimal use of DNA methylation changes and be able to modulate DNA methylation at specific targets for early disease intervention.
Meet the experts
Per Hoffman | Head of Commercial Operations Genomics at Life & Brain Genomics
Per Hoffman has been involved in the field of genomics since 2005, the time when the first microarrays for GWAS just became available. Since then, his work has contributed to the identification of new genes and loci in a variety of diseases and traits, among them dyslexia, bipolar disorder, schizophrenia, cleft lip/palate, myeloma and psoriasis. The main focus of Per’s current work is the application and adaption of genomic technologies for the investigation of the genetic basis underlying multifactorial diseases and traits. This includes microarrays, next- and third-generation sequencing, as well as laboratory automation, IT-infrastructure, process management and bioinformatics.
Robert Hillary | Research Fellow at University of Edinburgh
Robert Hillary is a postdoctoral research fellow based at the Institute of Genetics and Cancer, University of Edinburgh (UK). He received his PhD working with Riccardo Marioni as part of the Wellcome 4-year Translational Neuroscience programme at the University of Edinburgh (2017-2021). His PhD thesis was awarded the Sir Kenneth Mather Memorial Prize from the Genetics Society and University of Birmingham (UK) for outstanding performance in population genetics. His interests lie in using multiomics analyses (including epigenomics, genomics and proteomics) and causal modelling approaches to understand biological mechanisms underlying complex disease states. His work also focusses on developing epigenetic predictors of human traits and testing their associations with health outcomes using data from large, population-based studies.
Jörg Tost | Director of Laboratory for Epigenetics and Environment at the Centre National de Recherche en Génomique Humaine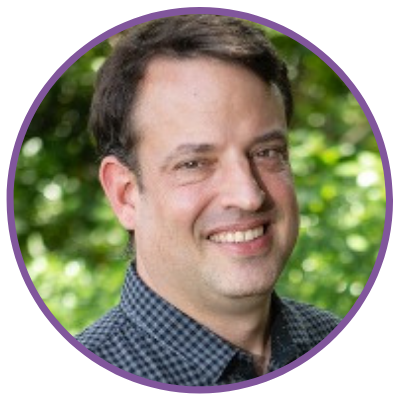
Jörg Tost received his PhD in Genetics from the University of Saarbrücken (Germany) in 2004 devising novel methods for the analysis of haplotypes and DNA methylation patterns. After a postdoctoral stay in the technology development department of the Centre National de Génotypage (Evry, France), he led the Epigenetics groups from 2006-2012, before becoming Director of Laboratory for Epigenetics and Environment at the Centre National de Recherche en Génomique Humaine (Evry, France). The laboratory is involved in the development and application of technologies to analyze DNA methylation, miRNAs and other epigenetic modifications quantitatively at high resolution at target loci and genome-wide using state-of-the-art sequencing technologies as well as the development of bioinformatic tools for the processing of such data. The laboratory has focused the analysis of epigenetic changes to neurodegenerative, autoimmune and inflammatory diseases as well as the alteration of the epigenetic profiles in function of environmental exposure. Jörg Tost is author or co-author of more than 197 publications and senior editor of the journal, Epigenomics.
For Research Use Only. Not for use in diagnostic procedures.
The opinions expressed in this interview are those of the interviewees and do not necessarily reflect the views of BioTechniques or Future Science Group.
This feature was produced in association with Illumina.