Counting synapses by algorithm
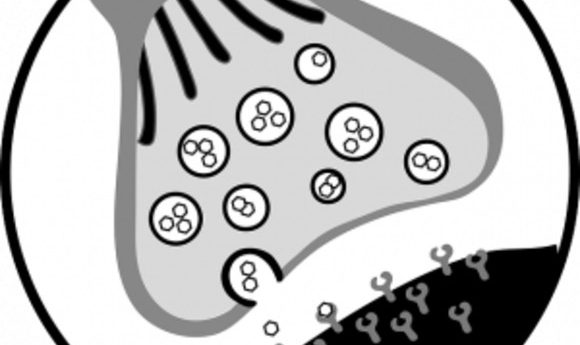
Several neuropsychiatric disorders are characterized by too many or too few synapses in key brain circuits. Now, a new machine learning algorithm should help advance studies of these diseases by counting the number of synapses in research samples.
The human brain, home to more than 80 billion neurons, hosts trillions upon trillions of synapses, the functional contacts by which neurons communicate with one another. This number matters: several studies have connected functional problems in the brain with too many or two few of these contacts.
“Many disorders like schizophrenia or autism spectrum disorder affect the number of synapses generated by neurons in the brain,” said Zhiping Pang, a neuroscientist who studies synaptic transmission and regulation at Rutgers University. “It is important for many studies to understand the exact number of synapses that are formed by neurons to help us better understand where the issue may be.”
Historically, counting synapses has been tedious work. Researchers traditionally used immunohistochemistry to label the puncta, or presynaptic contacts of neurons, and after using thresholding techniques, manually counted the labeled areas. But given the variability in results introduced by different cell culture techniques, antibody labeling, and human error, Pang thought there had to be a better—and more accurate—way to quantify synapses.
“With large analyses, it can take your student or post-doc forever to count the synapses. And you can’t always be sure that you counted them all. We wanted to consider synapse quantification more objectively,” he said. To do so, he and his colleagues combined a watershed thresholding technique with a machine learning algorithm to create a high-throughput, fully-automatic synapse-counting program called Intellicount. The team spent more than a year optimizing and validating the algorithm with data from previously published studies.
“This program lets the computer decide what is the synapse based on the algorithm’s learning experience,” Pang explained. “And after being trained on large data sets from both mouse and human neurons, the program is very good at capturing synapses with both low-intensity and high-intensity signals. Because of the limitations of optical imaging, it can be difficult to define the boundary of synapses. But the machine learning in our program helps trace out the real boundary of the synapse puncta as well.”
While Pang cautions that Intellicount is still a “work in progress,” he is making the program freely available to the research community to gain further insights on how to improve it. Interested researchers can find information about licensing the program on the Rutgers University website.