Single-cell analysis reveals how blood cells form
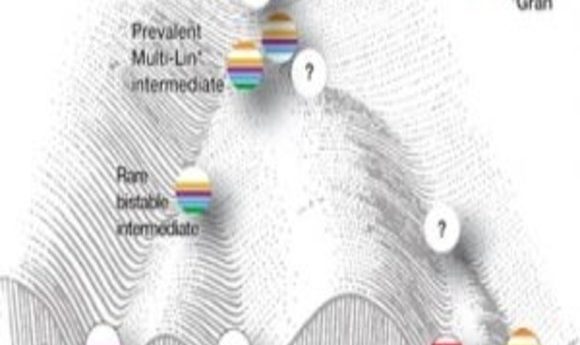
A recent study showing that developing blood cells are caught in a tug of war between competing gene regulatory networks has generated controversy, highlighting the challenges in analyzing single-cell data.
Model of mixed-lineage transition states underlying myeloid cell-fate determination (2).
Technologies providing genome-scale molecular information at single-cell resolution have offered unprecedented power for the systematic investigation of cellular heterogeneity. In particular, single-cell RNA sequencing has emerged as a revolutionary technique offering an unbiased strategy for identification and characterization of distinct cellular populations through the quantification of all transcripts expressed in a single cell (1). Moreover, specialized computational methods for processing and analyzing single-cell data are now making it possible to observe rare and intermediate cell states that cannot be resolved at the population level.
A study published last fall in Nature combined single-cell RNA sequencing with a new analytic tool called iterative clustering and guide-gene selection (ICGS) to delineate hierarchical genomic and regulatory states that culminate in the specification of neutrophils and macrophages in mice (2).
The findings showed that developing blood cells are caught in a tug of war between competing gene regulatory networks before deciding their fates. “It is somewhat chaotic, but from that chaos results order,” said study co-author Harinder Singh from Cincinnati Children’s Hospital Medical Center. “It’s a basic finding that helps us address a fundamental question of developmental biology: What are the natures of the intermediate states and the networks of regulatory genes that underlie cell-type specification.”
Dynamic Instability
A major challenge for developmental biologists is delineating hierarchical cellular states, including rare intermediates and the networks of regulatory genes, that orchestrate cell-type specification. Although a bistable gene regulatory network has been proposed to regulate the specification of either neutrophils or macrophages, the nature of the transition states and the underlying dynamics of the cell-fate determinants remain elusive.
Singh and his collaborators set out to address these questions using single-cell RNA sequencing. The problem was that their analysis of the single-cell data using six independent computational approaches resulted in the varied delineation of cellular states and intermediates.
Unable to make sense of their single-cell gene expression data, the researchers decided to develop a new analysis approach, called ICGS, which would use pairwise correlation of dynamically expressed genes and iterative clustering with pattern-specific guide genes to delineate coherent gene-expression patterns. This new method resolved nine hierarchically ordered cellular states, generating a refined order of discrete myeloid cell states independent of, but consistent with, prior knowledge.
Although the scientists still don’t understand exactly what steers the cell toward its eventual fate, their work does suggest that simultaneous expression of counteracting regulatory gene network components manifests as dynamic instability, resulting in mixed-lineage states that are necessary to prime newly forming cells for that decision. The data also suggest that the influence of two simultaneous and counteracting gene networks generates internal oscillations that are eventually stabilized by unknown mechanisms to generate one of two different cell fates.
“What myeloid cells are and how they develop from mesodermal and committed hematopoietic precursors is of interest not just to those studying the formation of normal blood cells in the bone marrow but also to developmental and disease biologists because of their presence and roles in many tissues during development, homeostasis, and resilience,” said study author Bruce Aronow of Cincinnati Children’s Hospital Medical Center. “Understanding their lineage development from the bone marrow context and the ability to further study this using in vitro models provided a foundation for studies that would have implications across many disciplines.”
Analyzing the Avalanche of Data
Aronow and Singh’s study offers just one of many models recently proposed to reconstruct cell lineages from single-cell RNA sequencing data analysis, according to Guo-Cheng Yuan, whose team at Harvard University focuses on developing computational biology methodologies for genomic data analysis and integration. “However, the results could vary depending on the specific experimental protocol and data analysis method,” he warned. “This is a general problem in the single-cell analysis field, in part because there are still a number of challenges in obtaining high-quality single-cell RNA sequencing and the subsequent data analysis and interpretation. A common practice in the field is to predict cell lineage based on gene expression similarity, but it is often difficult to directly validate such predictions rigorously.”
While the authors of the Nature study are aware that the mixed-lineage intermediates they described have generated some controversy, Singh is quick to point out that such intermediates were identified and characterized in hematopoiesis well before the advent of single-cell RNA sequencing.
Aronow also acknowledged that the study could have given different insights versus other single-cell analysis approaches. “With regard to our ability to identify, catalogue, and inter-relate the patterns of variation that we observed, the ICGS approach was sufficient to be much more confident about the true transcriptome of each cell as compared to data from the newer, lower-coverage technologies,” he said.
To overcome this issue, many researchers are hoping for greater standardization for single-cell analyses in the future. But it might be too early in the game for that. “I do feel overall that the field of single-cell sequencing is in rapid ferment to the point I’m not sure that standardization efforts would be fruitful,” said Jim Kent, a research scientist at the University of California, Santa Cruz. “It’s frustrating to a lot of people I understand,” Kent added. “Hopefully methods that are cheap enough and good enough to standardize will emerge in the next year or two. People are pressing the technology so hard now though that it is getting rapidly cheaper and better, and this trend is probably more important than standardization at this phase.”