Proteomics and “The Hitchhiker’s Guide to the Galaxy”: an interview with Grant Brown and Brandon Ho
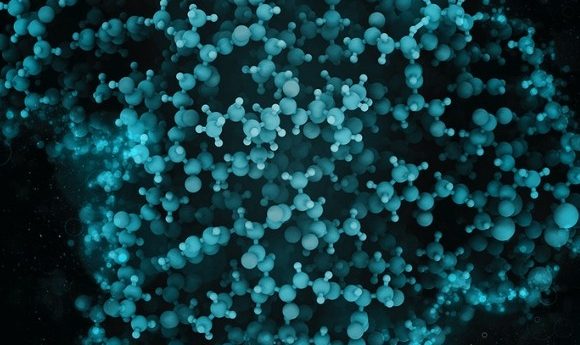
In this interview with Grant Brown and Brandon Ho from Toronto University (Canada), they discuss their research on determining the protein abundance of a yeast cell and question just how close we are to determining the protein abundance in human cells.
Professor Grant Brown runs a lab in the Department of Biochemistry at the University of Toronto (Canada). Grant received his PhD in Molecular Biology from UCLA (CA, USA) where he worked in Dan S. Ray’s lab studying DNA replication in the trypanosomatid C. fasciculata. His postdoctoral fellowship was at the Johns Hopkins University School of Medicine (MD, USA) with Tom Kelly where he studied regulation of S phase in fission yeast. He has been at the University of Toronto since 1999, an associate professor since 2004 and a professor since 2011.
3922
Brandon Ho is a 4th year PhD student in Grant’s lab in the Department of Biochemistry at the University of Toronto (Canada).
3921
Please can you give us an overview of your research?
Brandon: We use cell biology, biochemistry, genomics and proteomics to study how cells respond to stress. In particular, we’re more interested in replication stress. Our most recent project was looking at how proteins are quantified in a cell. Quantifying the abundance of proteins in a cell is important because they are one of the primary functional units in a cell. We’re interested in looking at how their abundance changes in response to stress – DNA damage, for example.
So what were the major findings of your research?
Brandon: It’s an overview of what the abundance is of any one protein in the cell. Providing this data set is, and already has proven to be, useful. We used the data to answer some fundamental questions about abundances of particular proteins in response to stress, like: what is the abundance of every single protein and how do they compare with one another? What is a low abundant protein compared to a high abundant protein?
Grant: For me, what was particularly interesting about the analysis that Brandon did is if you consider how scientists think about cells, a lot of what we spend our time doing is thinking about how things change in response to different kinds of environment: they can be internal or external; they can have different genetic backgrounds; different genotypes.
The way this has been traditionally approached is to look at mRNA. The reason for that is that the tools for looking at mRNA are better than the tools for looking at proteins in a genome-wide or proteome-wide basis but the bottom line is that usually what we’re interested in isn’t the level of mRNA; it’s the level of protein.
It’s pretty clear that variation in mRNA number doesn’t account for a lot of the variation in protein number. So we thought this was a nice opportunity to really address what gene expression and protein level is like in a cell and once you have a starting point, then you can start to ask questions about how protein expression changes.
So, the bulk of Brandon’s work was defining the protein abundance, but then he was also able to start on how you can then use that information to look at how the environments of protein change across the entire proteome. Most of the functionality in the cell is carried out by proteins. So, when people want to figure out what the inner workings of a cell are and how that changes, I think they really are talking about how the proteins are changing.
Which techniques proved particularly useful to you and why?
Brandon: The technique used in our research was a computational analysis so I guess the normalization scheme and the conversion to all molecules per cell is at the foundation of our story. Why it was so useful is that it’s a relatively simple but also intuitive measurement and conversion. In terms of the wet lab work that was done in the literature; there were so many orthogonal approaches to quantifying the same thing, which is protein abundance in the cell. This allowed us to do cross comparisons and having 21 data sets available to us really enabled us to obtain numbers that were more confident.
Grant: There were two things that made the study interesting and successful. First, there were a lot of good data sets that existed in the literature and the yeast model system is a little bit unique in that certainly in mammalian cells there are a lot of protein abundance data sets but they are restricted pretty much exclusively to mass spectrometry studies, whereas in yeast there are complementary analyses that have been done with GMT fusion proteins either by flow cytometry or microscopically. Then, there’s also an amazing data set where Jonathan Weissman’s group did an affinity purification western blot of each protein in the proteome.
There are these three independent approaches that you can use as a complementary evaluation of the other so you’re not relying on any one technique to drive your datasets. That leant a lot of power to the analysis.
Brandon figured out a simple to implement yet powerful approach to normalizing all these data sets that are expressed in wildly different units, to enable comparison between them. That’ll be useful in importing that kind of analysis into other systems. I think this computational approach could be readily applied to systems beyond the yeast model.
Do you think this research can now be applied to determine protein abundance in human cells or mammalian cells?
Brandon: In short, yes.
I think the technique and the method to do all of those conversions is pretty straight forward and can be implemented in other systems. At least one of the obstacles in human cells is that there are so many different cell types, different protein expressions and profiles within those cells. So to try to gain a sense of what is a normal human cell would be difficult but in theory you could do the same sort of analysis for particular cell types. Also, there is the lack of comprehensive data sets out there for human cells so this analysis could be done if there were data sets that were more complete or available.
What tips would you give to a researcher hoping to work in this field?
Grant: I can think of a few things. One thing that was particularly useful was that Brandon has a very strong talent for data visualization and doing a lot of exploratory data analysis. Looking at different kinds of visualizations can be really instructive when you’re dealing with large data sets and so that was really helpful in guiding us.
Quite early on, we approached Anastasia Baryshnikova, one of the co-authors on the study, who has a very strong background in computational biology. We started talking to her quite early and she was very helpful in guiding us so it’s always good to seek out complementary expertise.
We initially posted a preprint on bioRxiv, which was super useful because it lead to a lot of strong and helpful feedback on the study.
It was also really useful going back and forth with a knowledgeable editor and really trying to shape the final product. So I guess I would give a shout out to both the ideas of posting a preprint on bioRxiv to get feedback from the community and engaging the editors of the journal that you ultimately want to end up publishing in.
What can this research be used for in the future and what direction are you going in now?
Brandon: We’ll use this for further exploratory analyses. There are other factors such as degradation rates or half-life of mRNA that we are ultimately interested in but we don’t really have any comprehensive data sets on those measurements yet. Once those come out in literature I think it’ll be interesting to continually add to the data set and merge other data sets for other measurements to really get a sense of what is going on in the cell at a proteomic and genomic level.
Grant: We’ve been getting a lot of requests for the underlying data sets and the analysis methodologies so I think this is already being deployed pretty actively in the community. I think one of the ways that it’s being deployed is the bigger and better your data set is, the more readily it can be used compared to other sorts of genome-wide, proteome-wide analyses; to answer questions such as: what’s the relationship between mRNA abundance and protein abundance? What’s the relationship between translation rates and protein abundance? What is the relationship between protein half-life and protein abundance?
All of these sorts of questions depend on having high coverage; high quality data sets so I think these data are going to be tremendously useful for the community.
We’re in the middle of working with the Saccharomyces genome database to get this information online so that it is more accessible to the community so I think that’ll result in it being used more.
We now have a good idea about what the abundance of a particular protein is across an entire population but now I think we’re in a position to start to look at how that varies from cell to cell because to me, the question of the variation between genetically identical cells is at the heart of many different aspects of biology.
I guess an easy way of thinking about it, anecdotally and scientifically, is that different people respond differently to the same kind of disease treatments and so the underlying basis of that is going to be interesting to explore.
Do you have anything else to add?
Grant: Do you know The Hitchhikers Guide to the Galaxy? (It’s an old British radio play). There’s a point in it where a super computer generates the answer to life, the universe and everything and the answer is 42. Coincidentally, when we added up our proteome numbers, the total number of proteins in a yeast cell is 42 million.