Caught on camera: invasive species monitored using satellite imagery and AI
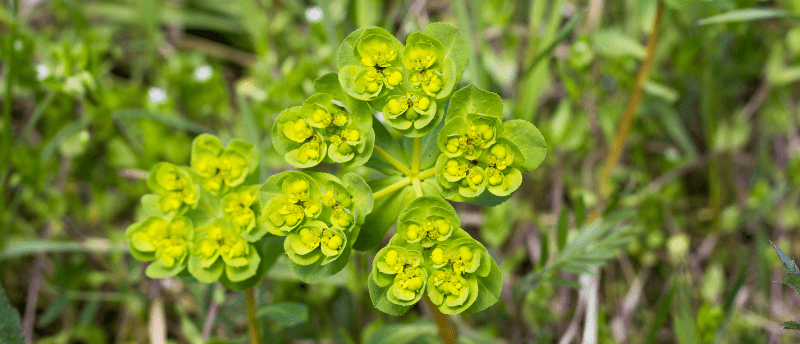
A recent study has demonstrated how convolutional neural networks can be used to identify and monitor invasive plant species… from space!
Three researchers from the University of Minnesota (MN, USA), David Moeller, Thomas Lake and Ryan Briscoe Runquist, have developed convolutional neural networks, a type of deep learning model, that can be trained to recognize certain objects in satellite images. This model was used to correctly identify the location of an invasive species – Euphorbia virgata, known colloquially as leafy spurge – from satellite images of the Twin Cities area (MN, USA). Invasive species pose an agricultural and economic threat; therefore, the detection and eradication of these species is of public importance.
Currently, to monitor invasive species, on-site field surveys are used to collect data such as population size, density and growth rate. This is time consuming and expensive and will not be able to keep up with the rapid growth and spread of invasive species. The development of an artificial intelligence (AI) model for detecting invasive species could provide an alternative, more efficient way of monitoring invasive species. Moeller commented, “Invasive species are more difficult than ever to manage and surveillance via satellites is a low-cost, rapid method for dynamically monitoring invasions.”
Researchers trained deep learning models to recognize Euphorbia virgata from satellite images. They used both rare high-resolution images and lower-resolution everyday images to test whether the model could monitor invasive plant growth and movement over time.
CRISPR-Combo combines gene activation and editing
Simultaneous gene activation and gene editing for different targets in plant genomes is made possible with CRISPR-Combo.
Their AI model identified Euphorbia virgata with greater than 96% accuracy from both higher-resolution and numerous lower-resolution satellite images of the Twin Peaks area. Another of the AI models that tracked images over time could predict the timing of plant emergence, flowering and senescence.
The study demonstrated that convolutional neural networks could be used to accurately detect an invasive species in Minnesota. Using lower-resolution images over time offers an opportunity to track invasive species rather than simply identifying them. In future, the researchers plan to track Euphorbia virgata growth in other parts of the USA over the last 35 years and predict how climate change might change this invasive species’ dispersal.
“Time series analysis is relatively new in this type of deep learning model so we were really excited to find that it worked so well at identifying leafy spurge populations,” commented Briscoe Runquist. “Deep learning allows scientists to discover patterns that would have been previously impossible to detect.”